“In the middle of every difficulty lies opportunity.” – Albert Einstein. This quote highlights the importance of tackling research data challenges in today’s world. As we move into 2024, dealing with ambiguity is more critical than before. Traditional methods often fail with imprecise data, making Fuzzy Set Analysis key for managing ambiguity.
[Short Notes] Fuzzy Set Analysis: Dealing with Ambiguity in Research Data
Understanding Fuzzy Set Analysis
Fuzzy set analysis is a powerful tool in research methodology that allows for the handling of ambiguous or imprecise data. Unlike traditional set theory, which deals with binary membership (an element either belongs to a set or it doesn’t), fuzzy set theory introduces the concept of partial membership. This approach is particularly valuable in social sciences, psychology, and other fields where data often resist clear-cut categorization.
In Simple Words
Imagine you’re sorting fruits into baskets. With regular sets, an apple goes in the “apple basket” and nowhere else. But what about a fruit that’s half-apple, half-pear? Fuzzy sets let you put it partially in both baskets. It’s like saying, “This fruit is 60% apple and 40% pear.” This helps when things aren’t black and white in research.
Why Fuzzy Set Analysis Matters
In real-world research scenarios, data often doesn’t fit neatly into predefined categories. Fuzzy set analysis provides a framework to deal with this ambiguity, offering several advantages:
- More accurate representation of complex phenomena
- Ability to capture nuanced differences in data
- Enhanced flexibility in data analysis and interpretation
- Better alignment with human reasoning and natural language
Did You Know?
Fuzzy set theory was introduced by Lotfi A. Zadeh in 1965. Initially met with skepticism, it has since become a cornerstone in fields ranging from artificial intelligence to climate science.
Applying Fuzzy Set Analysis in Research
Researchers can apply fuzzy set analysis through various methods:
- Fuzzy Clustering: Grouping data points with varying degrees of membership to multiple clusters.
- Fuzzy Logic Systems: Creating rule-based systems that can handle imprecise inputs.
- Fuzzy Regression: Analyzing relationships between variables when boundaries are unclear.
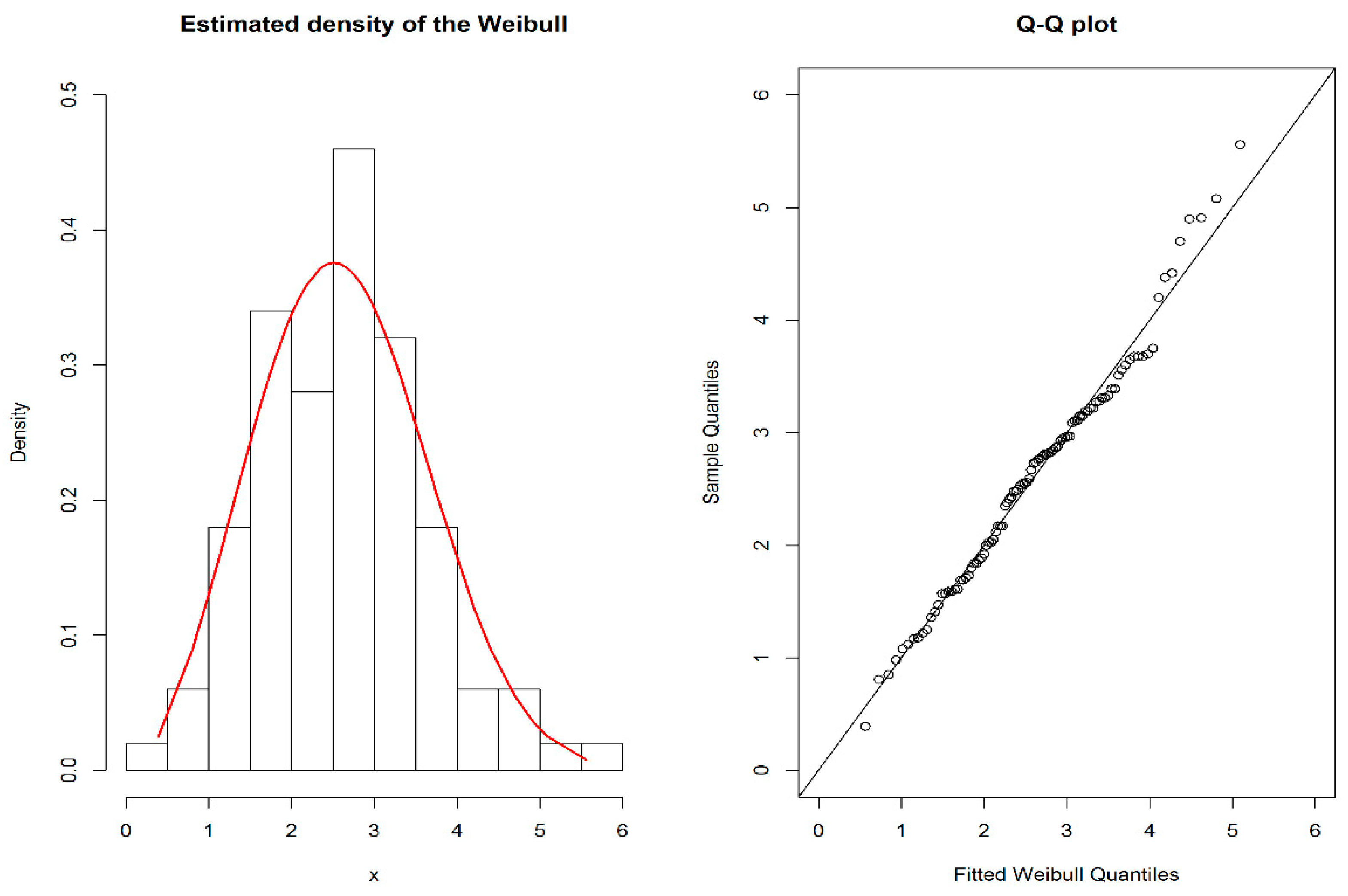
Figure 1: Example of a Fuzzy Set Membership Function. Source: MDPI (Open Access)
Comparative Analysis: Traditional vs. Fuzzy Set Approaches
Aspect | Traditional Set Theory | Fuzzy Set Theory |
---|---|---|
Membership | Binary (0 or 1) | Continuous [0, 1] |
Boundaries | Crisp | Gradual |
Handling Ambiguity | Limited | Extensive |
Applicability to Real-world Scenarios | Moderate | High |
“The world is not black and white but only shades of gray.” – This sentiment, often attributed to W. Edwards Deming, encapsulates the essence of fuzzy set theory in research.
Challenges and Considerations
While fuzzy set analysis offers numerous benefits, researchers should be aware of potential challenges:
- Increased complexity in data interpretation
- Need for specialized software and expertise
- Potential for subjectivity in defining membership functions
- Balancing precision with the advantages of fuzzy logic
Enhance Your Research with Editverse
Navigating the complexities of fuzzy set analysis? Editverse.com offers expert research consultancy, manuscript writing, and publication support services. Our team of specialists can help you apply fuzzy set methodologies effectively, ensuring your research captures the nuances of complex data. From conceptualization to publication, Editverse is your partner in advancing cutting-edge research.
Future Directions
The field of fuzzy set analysis continues to evolve, with promising applications in:
- Big Data Analytics
- Machine Learning and AI
- Decision Support Systems in Healthcare
- Environmental and Climate Science Modeling
Conclusion
Fuzzy set analysis represents a paradigm shift in how researchers approach ambiguous data. By embracing the complexity and nuance inherent in many research domains, this methodology opens new avenues for insight and understanding. As research continues to grapple with increasingly complex phenomena, the tools and perspectives offered by fuzzy set analysis will undoubtedly play a crucial role in advancing scientific knowledge.
References
- Zadeh, L. A. (1965). Fuzzy sets. Information and Control, 8(3), 338-353.
- Ragin, C. C. (2000). Fuzzy-Set Social Science. University of Chicago Press.
- Zimmermann, H. J. (2010). Fuzzy set theory. Wiley Interdisciplinary Reviews: Computational Statistics, 2(3), 317-332.
Example – Fuzzy Set Analysis in Global Health Research: A 131-Country Study
Let’s examine a concrete application of Fuzzy Set Qualitative Comparative Analysis (fsQCA) in global health research, based on the following study:
Reference Study
Çakmaklı, D., Booysen, F. & Bohler-Muller, N. A fuzzy set qualitative comparative analysis of 131 countries: which configuration of the structural conditions can explain health better?. Int J Equity Health 18, 46 (2019).
Access the full paperStudy Overview
This research applies fsQCA to investigate how different combinations of structural conditions across 131 countries explain variations in population health outcomes.
Key Concept: Fuzzy Set Qualitative Comparative Analysis (fsQCA)
fsQCA is a method that combines qualitative and quantitative analysis. It allows researchers to identify complex causal patterns and multiple pathways to an outcome, considering that different combinations of conditions may lead to the same result.
Application of Fuzzy Set Principles
- Calibration: The study calibrates raw data into fuzzy set membership scores between 0 and 1, allowing for degrees of membership in sets.
- Complex Causality: fsQCA acknowledges that multiple combinations of conditions can lead to the same outcome, aligning with the complexity of real-world health scenarios.
- Equifinality: The method recognizes that different paths can lead to the same outcome, capturing the diverse ways countries achieve good health outcomes.
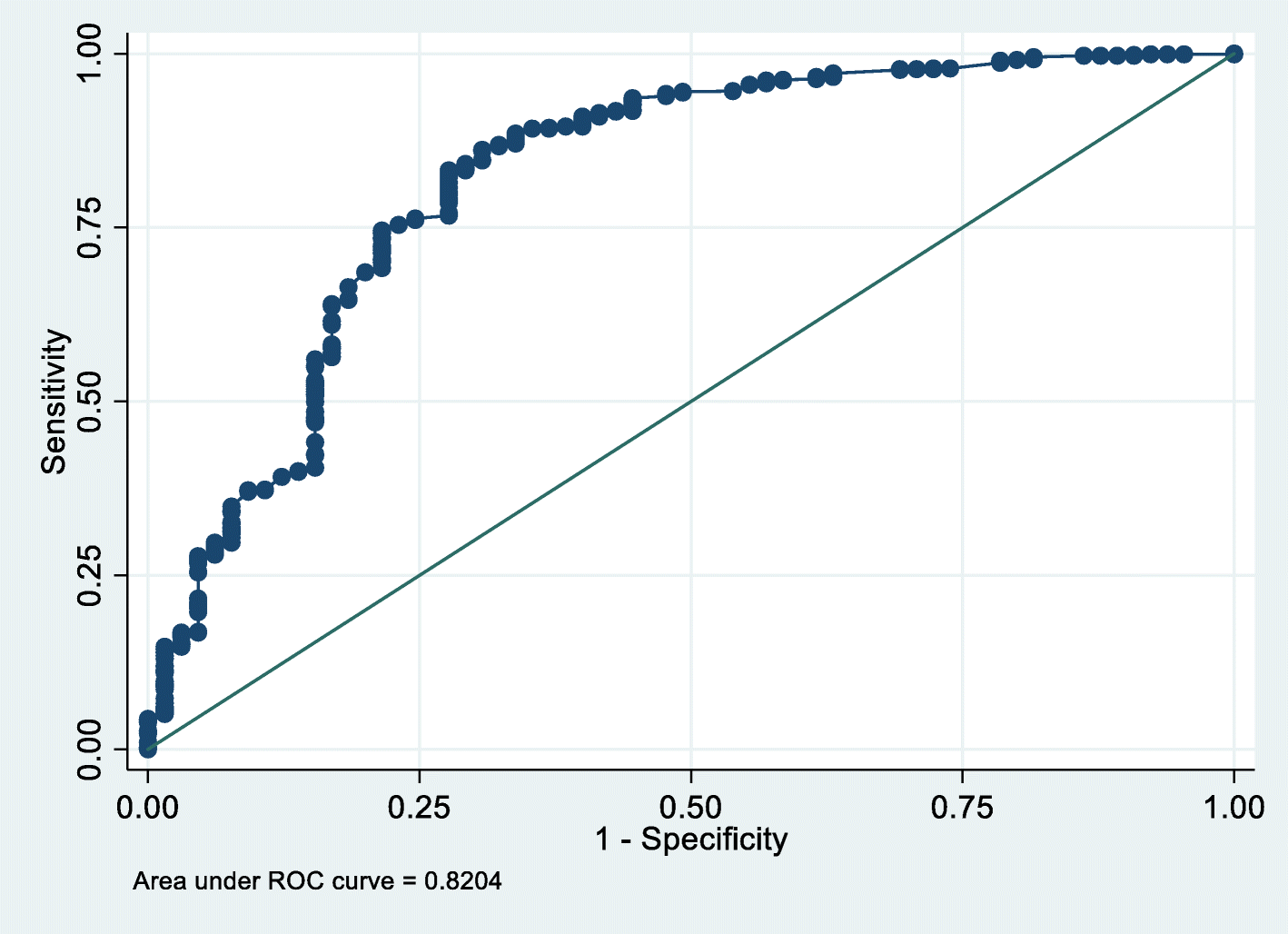
Figure 1: Calibration of the outcome and causal conditions. Source: Çakmaklı et al. (2019), Open Access
Key Findings Through Fuzzy Set Analysis
- The study identified five different configurations of structural conditions that explain good health outcomes.
- These configurations involve combinations of economic development, income inequality, political rights, civil liberties, and ethnic fractionalization.
- The analysis revealed that no single condition is necessary or sufficient for good health outcomes, highlighting the complex nature of health determinants.
Fuzzy Set Interpretation
The fsQCA approach allowed researchers to:
- Identify multiple pathways to good health outcomes, reflecting real-world complexity.
- Consider degrees of membership in sets (e.g., degrees of economic development) rather than binary classifications.
- Uncover combinations of conditions that traditional regression analyses might miss.
Advantages of fsQCA in This Study
- Captures complex interactions between structural conditions and health outcomes
- Allows for the identification of multiple sufficient paths to good health
- Provides insights into both developed and developing country contexts
- Offers a nuanced understanding of how different factors combine to influence health
“The fsQCA method enabled us to uncover complex causal patterns in global health outcomes that might be overlooked by traditional statistical methods. This approach recognizes that the path to good health is not one-size-fits-all across different countries and contexts.” – Interpretation based on the study’s methodology and findings
Conclusion
This study demonstrates the power of fuzzy set analysis in global health research. By using fsQCA, the researchers were able to identify multiple pathways to good health outcomes across diverse countries, accounting for the complex interplay of economic, political, and social factors. This nuanced approach provides valuable insights for policymakers and health professionals working to improve population health in varied global contexts.
The research world is changing, bringing new challenges in understanding complex data. Fuzzy Set Analysis provides a strong way to handle uncertainty. It helps researchers get valuable insights from unclear data. This method is used in various areas, like new work behaviors, company structures, and green practices in small businesses, as seen in 13 studies from top journals1. Learning to use Fuzzy Set Analysis can open new doors in your research.
As you explore this topic, remember the big role Fuzzy Set Analysis has. It helps us deal with today’s complexities and make better decisions in different fields. Using these strategies can make your research clearer and more impactful.
Key Takeaways
- Fuzzy Set Analysis is crucial for managing ambiguity in 2024 research data.
- Traditional methods find it hard with complex and unclear data.
- Research includes insights from top journals.
- Fuzzy Set Analysis supports new practices in many areas.
- Handling ambiguity well can improve decision-making.
Understanding Fuzzy Set Analysis
Fuzzy Set Analysis is a key tool for tackling complex issues in research. It moves beyond traditional yes or no thinking, offering a new way to handle unclear data. This method lets researchers like you deal with uncertainty quantification in different datasets.
A recent study showed how Fuzzy Set Analysis works well with life expectancy data from 131 countries. It found that things like governance, education, wealth, and health greatly affect life expectancy. Often, a mix of these factors leads to lower life expectancy2. This shows how ambiguity management helps us better understand health trends worldwide.
This study highlights the need for a method that handles unclear and complex data. Fuzzy logic is great for this, offering a structured way to look at these issues. It helps in making better decisions and improves the accuracy of your research.
Fuzzy logic is useful in many areas, from economics to medical diagnostics, dealing with unclear data3. The methods of fuzzy set analysis help make sense of unclear or partial data. This makes your findings more reliable.
Fuzzy set analysis is also useful in studying workplace dynamics and innovation. It helps understand how companies work together and learn from each other. In looking at how workplaces learn, fuzzy methods have been key in linking different factors together. This adds a lot to our knowledge in organizational psychology1. It’s a powerful tool for researchers dealing with complex social science topics.
What is Fuzzy Logic?
Fuzzy logic is a new way to think about things that aren’t clear-cut. It was first talked about by Lotfi Zadeh in 1965. Unlike traditional logic, which only sees things as true or false, fuzzy logic uses partial truths between 0 and 1. This makes it great for handling complex situations.
This idea has led to many Fuzzy Logic Applications. You can find it in everything from industrial systems to artificial intelligence.
The Origins of Fuzzy Logic
Zadeh’s work, “Fuzzy Sets,” introduced this new logic in 1965. At first, many in the West didn’t get it. But in the 1980s, Japan saw its potential and made big strides with it. Now, fuzzy logic helps in many areas, like aerospace and making business decisions45.
Comparison with Traditional Logic
Traditional logic splits things into just true or false. But fuzzy logic is more like how we really think, dealing with gray areas. It’s better for problems with unclear or missing info.
Fuzzy logic can give right answers even with bad data. It’s used in things like understanding feelings, controlling traffic, and even in smart systems like IBM’s Watson46.
Importance of Ambiguity Management
Ambiguity in research data is a big deal for making good decisions. It’s key to understand its effects to make better choices, especially when things are unclear. When data is incomplete or unclear, it’s hard to predict outcomes. This shows why we need good Ambiguity Management strategies.
Defining Ambiguity in Research Data
Ambiguity means having many possible meanings because of unclear or missing info. This is common in research, especially when opinions play a big role. To get useful insights, research must tackle ambiguity head-on. Imprecision Mitigation Strategies help make data clearer. For example, qualitative metasynthesis research looks into people’s experiences with certain conditions¹.
Understanding conditions like Mild Cognitive Impairment (MCI) shows how tricky it can be. The unclear nature of these conditions makes analyzing them tough².
The Role of Uncertainty in Decision Making
Uncertainty is key in making decisions. When we don’t have much info, it can greatly affect what we decide. Ambiguity aversion is when we prefer known risks over unknown ones. This can lead to worse decisions because uncertainty can mess with how we see risks and impact how well organizations do over time³.
Knowing the value of information (VoI) is crucial. It’s about how new, clearer info changes expected outcomes. This shows how clear info can really help in making better decisions³.
Strategies like scenario planning and adapting help us deal with uncertainty. These methods let us work with the unknown and still make informed choices. Using fuzzy logic can also help accept gradual truths, leading to stronger decisions despite ambiguity7.
Fuzzy Set Analysis: Dealing with Ambiguity in 2024 Research Data
Fuzzy Set Analysis is key in handling the complex and unclear parts of 2024 Research Data. As more research papers come out each year, the need for Imprecise Data Handling grows. Many studies focus on fuzzy logic to understand unclear situations8. By comparing different ways to make decisions with fuzzy sets, we see the many ways to look at data today.
Now, using intuitionistic fuzzy sets in making decisions is becoming more common8. This shows how useful these methods are for dealing with unclear situations. Also, fuzzy time series forecasting is being used more in different fields. This shows its value in real situations. It lets researchers use fuzzy set analysis in many areas with confidence.
More people are using T-spherical fuzzy power aggregation operators in making decisions9. This change shows a better understanding of how reliable and uncertain data can be. Statistics are key in figuring out the uncertainty in group decisions. This proves the fuzzy set ideas work well in real life.
By combining these new ideas, researchers can better understand Research Data and handle Imprecise Data Handling well. As Fuzzy Set Analysis grows, its importance in solving today’s research challenges is clear. This supports its wider use in both school and real-world settings.
Application Area | Statistical Insight |
---|---|
Fuzzy Time Series Forecasting | It’s being used more in various sectors because it’s flexible and works well. |
Decision-Making Algorithms | Studies show it’s good at dealing with uncertainty. |
Intuitionistic Fuzzy Sets | More people are using it for complex data. |
Components of Fuzzy Set Analysis Systems
Fuzzy set analysis systems have key parts that help manage uncertainty well. Each part has a special role in changing and handling data. This makes decisions better in many areas.
Fuzzy Sets and Their Membership Functions
Fuzzy sets are special because they let things belong to more than one set at once. This is shown by membership functions. These functions show how much something belongs to a set, from 0 to 1.
There are different types of membership functions like triangular, trapezoidal, sigmoidal, and Gaussian. Each type is chosen based on the problem being solved10.
Fuzzification, Inference, and Defuzzification Processes
The first step, fuzzification, turns clear data into fuzzy values. This helps the system deal with uncertainty better. Then, inference uses fuzzy rules to make new conclusions from the fuzzy sets.
After that, defuzzification turns fuzzy outputs back into clear values. This makes decisions easier. These steps are useful in many areas, from healthcare to artificial intelligence1110.
Applications of Fuzzy Set Analysis in Various Fields
Fuzzy set analysis is used in many areas, showing its power in dealing with uncertainty. It helps make better decisions. In fields like Control Systems and Consumer Electronics, fuzzy logic makes things work better and makes users happier.
Utilizing Fuzzy Logic in Control Systems
In Control Systems, fuzzy logic is key for handling unknowns and changing conditions. For example, it’s used in HVAC systems to make them work better. It adjusts to temperature and how many people are around, making things more comfortable and saving energy.
It also plays a big part in car technology. It helps with things like automatic brakes and keeping cars stable, using data from sensors.
Fuzzy Set Analysis in Consumer Electronics
In Consumer Electronics, fuzzy logic is seen in things like smart washing machines. These machines change their cycles based on what you want, like how much laundry you have and what it’s made of. This makes sure your clothes get clean well, saves water and energy, and makes users happy.
Studies show that these smart products do a better job than old-fashioned ones. For more info on how fuzzy logic changes things, check out this research article12.
Soft Computing Techniques and Their Relevance
Soft Computing Techniques are key for solving complex real-world problems. They help manage uncertainty and vagueness in fields like software maintenance and system optimization. Over 80% of the cost in software development goes to maintenance, showing how crucial these strategies are13.
Understanding Soft Computing Techniques
Approximate Reasoning is a big part of soft computing. It helps build strong Fuzzy Decision Support Systems. Researchers looked at 56 papers and 21 conference talks on predicting software maintainability13. They found that change maintenance effort and the maintainability index are key metrics.
Class-level product metrics are often used to predict software maintainability. This shows where more research is needed.
Approximate Reasoning Methods in Research
Soft computing is used in many engineering fields. A review found that 8 out of 45 sources were in the IEEE Transactions on Fuzzy Systems14. Fuzzy logic has made systems more efficient, especially in unmanned aerial vehicles.
Using fuzzy logic and approximate reasoning improves accuracy and functionality across sectors.
Imprecision Mitigation Strategies with Fuzzy Logic
In the world of research and decision-making, Imprecision Mitigation Strategies using fuzzy logic are key. They help us deal with the many uncertainties we face. By using fuzzy decision support systems, we can better understand and manage Uncertainty Quantification.
This is especially useful in areas like health, where making accurate decisions is crucial. For example, fuzzy logic has improved how we handle health data, making it more reliable15.
Fuzzy set theory (FST) is also vital in many areas, such as consumer electronics and artificial intelligence16. It helps in making decisions and predicting market trends. This is very useful when you need to look at things from different angles.
Fuzzy logic is flexible and can handle complex decisions well. It uses tools like fuzzy graphs to make analyzing uncertain data easier. This is especially useful in fields like medical diagnosis and imaging16.
Future Trends in Fuzzy Set Analysis
The future of fuzzy set analysis is looking bright as researchers dive into new methods and uses. We’ll see more fuzzy logic in decision-making, especially in different sectors. This will bring new ways to solve complex problems.
Anticipated Developments in 2024 and Beyond
In 2024, fuzzy optimization will become more popular. It’s already helping in many areas like solving network problems, improving manufacturing, and streamlining supply chains. This will lead to new research breakthroughs17.
Pythagorean fuzzy sets (PFS) are bringing new ideas to fuzzy logic. They help deal with uncertainty better18.
New tools, like advanced sorting for Pythagorean fuzzy sets, will change how we handle uncertain data. This fits with ongoing research on making better decisions in various industries17. Also, new strategies for solving complex problems show how fuzzy logic can be a strong tool17.
Fermatean Fuzzy Sets (FFS) are gaining attention for their ability to handle uncertain information. They could become more common in decision-making and studying complex systems18. Researchers are working hard to apply FFS in real situations, showing the ongoing need for new ideas in fuzzy set analysis18.
Looking ahead, we’ll see more studies on how fuzzy set analysis can help understand and fix differences in life expectancy across countries. Things like good governance, education, and healthcare are key to solving these issues19.
Research is opening up new ways to use Pythagorean fuzzy Multi-objective Optimization in fields like product development and material identification17. As we move forward, embracing fuzzy logic will be key to tackling today’s challenges. This ensures fuzzy set analysis stays a crucial tool for managing research data in the future.
Conclusion
Fuzzy Set Analysis is key in handling unclear data, especially as research gets more complex in 2024 and later. It combines qualitative insights with hard numbers to make sense of data that’s hard to analyze. This method boosts your ability to make smart choices, even when your data is unclear.
Using fuzzy logic gives a deeper look into complex human systems. Studies show it helps in tasks like sorting brain MRI images and finding odd data points in big databases20. As fuzzy logic grows, it’s set to get even better, bringing new tech to improve how we analyze data.
Fuzzy Set Analysis will keep being a must-have in your research tools. It helps you find clear actions from unclear data. For more on how fuzzy logic changes data analysis, check out resources on the FCM algorithm and its impact on analysis and clustering21. These resources also show the huge amount of data we deal with daily, making it crucial to have good data management methods.
FAQ
What is fuzzy set analysis?
How does fuzzy set analysis help in decision-making?
What are the key components of fuzzy set analysis systems?
Can you give examples of fuzzy logic applications?
What role do soft computing techniques play in fuzzy set analysis?
How does fuzzy set analysis handle imprecise data?
What future trends can we expect in fuzzy set analysis?
Source Links
- https://www.cambridge.org/core/journals/spanish-journal-of-psychology/article/fuzzyset-qualitative-comparative-analysis-fsqca-in-organizational-psychology-theoretical-overview-research-guidelines-and-a-stepbystep-tutorial-using-r-software/D559DE9DB4297F6F895C4B8424600EBA
- https://www.ncbi.nlm.nih.gov/pmc/articles/PMC11133931/
- https://www.linkedin.com/pulse/what-fuzzy-logic-aionlinecourse-fnj2c
- https://www.investopedia.com/terms/f/fuzzy-logic.asp
- https://www.geeksforgeeks.org/sentiment-analysis-using-fuzzy-logic/
- https://www.spiceworks.com/tech/devops/articles/fuzzy-logic/
- https://fastercapital.com/content/Fuzzy-logic–Embracing-Ambiguity–Subjective-Probability-and-Fuzzy-Logic.html
- https://www.aimspress.com/article/doi/10.3934/math.2024272
- https://www.nature.com/articles/s41598-024-68371-1
- https://www.linkedin.com/pulse/what-fuzzy-set-theory-aionlinecourse-odbfc
- https://www.ncbi.nlm.nih.gov/pmc/articles/PMC10376790/
- https://www.journal-fea.com/
- https://ar5iv.labs.arxiv.org/abs/2209.10131
- https://www.ieee-jas.net/en/article/doi/10.1109/JAS.2024.124530
- https://www.ncbi.nlm.nih.gov/pmc/articles/PMC8500271/
- https://www.nature.com/articles/s41598-023-43922-0
- https://www.ncbi.nlm.nih.gov/pmc/articles/PMC10587483/
- https://link.springer.com/article/10.1007/s10462-024-10761-y
- https://www.ncbi.nlm.nih.gov/pmc/articles/PMC5778742/
- https://link.springer.com/article/10.1007/s00521-024-09994-3
- https://journalofbigdata.springeropen.com/articles/10.1186/s40537-019-0206-3