As we move forward in the world of cross-sectional research, it’s key to know the best practices and the pitfalls we might face in 2024 and 2025. These studies help us spot trends, attitudes, and links in certain groups over a set period. We aim to share insights that boost our research quality by avoiding mistakes like unclear questions, wrong sampling, and incorrect data analysis. By following these best practices, we can make our research more trustworthy and reliable. This leads to better decisions in our fields. For more insights, check out a study on how technology is changing our world here1.
Cross-sectional Research: Best Practices and Pitfalls to Avoid in 2024-2025
Cross-sectional Research: Navigating Best Practices and Pitfalls
Introduction
Cross-sectional research remains a cornerstone of many scientific disciplines in 2024-2025, offering valuable insights into population characteristics at a specific point in time. This guide explores the latest best practices, common pitfalls, and emerging trends in cross-sectional research methodologies.
Key Advancements in 2024-2025:
- Integration of AI and machine learning for data analysis and pattern recognition
- Enhanced digital data collection methods for broader and more diverse sampling
- Improved statistical techniques for handling large-scale, complex datasets
- Greater emphasis on reproducibility and open science practices
1. Best Practices in Cross-sectional Research
Adhering to these best practices ensures the validity, reliability, and impact of cross-sectional studies in 2024-2025.
Key Best Practices:
- Utilize AI-powered sampling techniques for more representative population coverage
- Implement rigorous data quality checks and cleaning procedures
- Employ advanced statistical methods, including machine learning algorithms, for data analysis
- Ensure transparency by pre-registering studies and sharing data and code
- Conduct thorough power analyses to determine appropriate sample sizes
- Use validated, culturally sensitive measurement tools
- Address potential biases through diverse research teams and external audits
Case Study: AI-Enhanced Sampling in Public Health Research
A 2024 global health study used AI algorithms to optimize sampling strategies, resulting in a more representative and cost-effective cross-sectional analysis of mental health outcomes across diverse populations.
2. Common Pitfalls to Avoid
Researchers should be aware of these potential pitfalls that can compromise the validity and generalizability of cross-sectional studies.
Key Pitfalls:
- Over-reliance on AI without human oversight, leading to algorithmic bias
- Neglecting the temporal limitations of cross-sectional data in causal inferences
- Inadequate consideration of confounding variables in complex datasets
- Misinterpretation of correlations as causal relationships
- Insufficient attention to selection bias in digital data collection methods
- Overlooking the impact of rapidly changing societal factors on data relevance
- Inadequate reporting of non-response rates and their potential effects
Case Study: Algorithmic Bias in Social Media Research
A 2025 study on social media usage patterns faced criticism for using AI-driven data collection methods that inadvertently excluded certain demographic groups, highlighting the need for human oversight in AI-assisted research.
3. Advanced Techniques in Cross-sectional Research
Emerging methodologies and technologies are enhancing the depth and breadth of cross-sectional studies in 2024-2025.
Advanced Techniques:
- Integration of real-time data from IoT devices for dynamic cross-sectional snapshots
- Use of natural language processing for analyzing open-ended survey responses
- Application of virtual and augmented reality for immersive data collection experiences
- Implementation of blockchain technology for secure and transparent data management
- Utilization of federated learning for privacy-preserving multi-site studies
- Employment of quantum computing for complex statistical analyses
4. Ethical Considerations
As cross-sectional research methods advance, new ethical challenges emerge that researchers must navigate carefully.
Key Ethical Considerations:
- Ensuring informed consent in AI-driven data collection processes
- Protecting participant privacy in large-scale, integrated datasets
- Addressing potential harm from AI-generated insights or predictions
- Balancing open science practices with data protection regulations
- Considering the societal impact of research findings, especially in sensitive areas
- Ensuring equitable representation in studies utilizing advanced technologies
Future Trends
Looking beyond 2025, several emerging trends are set to further transform cross-sectional research methodologies.
Emerging Trends:
- Integration of neuroimaging data in large-scale cross-sectional studies
- Use of AI-powered digital twins for predictive modeling in population studies
- Implementation of quantum machine learning for ultra-complex data analysis
- Development of global, real-time cross-sectional monitoring systems
- Incorporation of genetic and epigenetic data in multidimensional analyses
- Advancement of cross-reality (XR) technologies for immersive data visualization
Conclusion
Cross-sectional research in 2024-2025 is characterized by the integration of advanced technologies, enhanced methodologies, and a growing emphasis on ethical considerations. While these advancements offer unprecedented opportunities for insights, they also present new challenges that researchers must navigate carefully.
Key Takeaways:
- AI and machine learning are revolutionizing sampling and analysis in cross-sectional studies
- Rigorous methodologies and ethical considerations are crucial for valid and impactful research
- Advanced techniques are expanding the scope and depth of cross-sectional insights
- Awareness of potential pitfalls, especially those related to new technologies, is essential
- The future of cross-sectional research lies in interdisciplinary approaches and ethical innovation
Key Takeaways
- Cross-sectional research identifies trends and associations in populations.
- Best practices enhance the validity and reliability of findings.
- Avoiding common pitfalls is crucial for effective studies.
- Understanding sampling methods improves research credibility.
- Flawed data analysis can compromise research integrity.
Understanding Cross-sectional Research
In our journey of Understanding Cross-sectional Research, we see it as a key type of study. It looks at data from a specific group at one point in time. This method gives us a full picture, which is great for learning about health issues in different groups.
This type of research has many benefits. It’s fast and can be less expensive than other ways to study things. We can look at many different things at once, which helps us learn more quickly. But, it’s important to know its limits, like not being able to show cause and effect.
These studies usually have two main types: descriptive and analytical. Descriptive studies just tell us about the group’s traits. Analytical studies look closer at how different things are connected. This makes it very useful in health studies and making health policies. In short, Cross-sectional Research is key to understanding health trends and making informed decisions2.
Importance of Sample Size Determination
In any successful study, picking the right sample size is key. If our sample is too small, we might get results that are unclear or biased. This can harm the trust in our study. We need to think carefully about how big our sample should be, based on our goals and what we expect to find. This often means doing detailed statistical analysis.
Many things affect how big our sample should be. These include the size of the population, how varied it is, and our confidence level. Knowing how these factors work together helps us find a good balance. By using smart sample size determination methods, we make our findings more reliable and reduce the chance of making mistakes.
Being thorough in planning helps us set clear goals and choose the right ways to collect data. In cross-sectional research, good planning leads to strong study methods. By following these steps, we make our studies better. This helps us make a bigger impact in the academic world in research design and doing it right3.
Data Collection Methods in Cross-sectional Research
Choosing the right Data Collection Methods is key for good Cross-sectional Research. We use tools like questionnaires, surveys, and interviews to get the info we need. These methods help us answer our research questions and focus on the right groups of people.
Using tested tools makes our data more reliable and accurate. It’s also important to be ethical and clear when working with people’s information. If we don’t collect data well, our findings might not be trustworthy. We need to make sure our methods match our research goals.
Creating questionnaires is a detailed process. We test them before use and might use other research methods to make them better. The Pew Research Center says following best practices in making questionnaires reduces bias and makes our questions clear reference4.
Seeing our data helps us understand complex results better. Using data-focused methods is also key when dealing with big data. These methods help us handle issues like accuracy and reliability in Cross-sectional Research5.
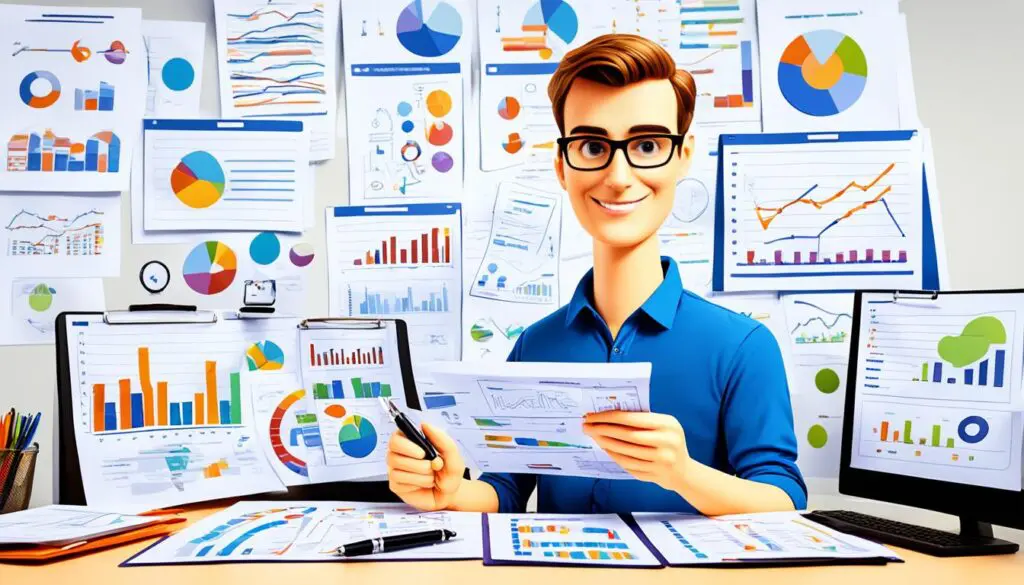
We work hard to get top-quality data, facing many challenges along the way. We must manage how we ask questions, the order of answers, and avoid biases. A smart approach in these areas leads to strong research methods. This way, we can make better decisions and stand out with careful research designs6.
Survey Design Best Practices
Creating a great survey is key to a successful study. We must make sure our questions are clear and to the point. Using objective language helps avoid confusion. A well-designed survey makes it easier for people to understand and answer.
Using different types of questions like open-ended, multiple-choice, and Likert scales gives us a wide range of data. This mix makes our survey comprehensive and engaging. Testing the survey first on a small group is crucial. It helps us fix any unclear questions before sharing it widely with everyone.
It’s important to follow rules for making questionnaires. Clear directions and correct grammar show we’re professional and help get better answers. It’s also key to avoid questions that show bias, which affects the study’s trustworthiness7.
- Keep questionnaires short to keep people interested.
- Give space for detailed answers.
- Use white space to make questions less crowded.
- Make closing instructions clear for easy finishing.
Analyzing the data is crucial to get useful results. We’ll connect questions, create scales, and use statistics to test our ideas. Following these best practices makes our study more reliable and valuable8.
In summary, good survey design reduces bias and gives us trustworthy insights. These insights are key to our research success.
Cross-sectional Research: Best Practices and Pitfalls to Avoid in 2024-2025
In cross-sectional research, it’s key to know the common pitfalls to get good results. We’ll look at the cross-sectional research landscape and the issues researchers might face.
Common Pitfalls in Cross-sectional Research
Not setting clear research goals is a big mistake. It can mess up the sampling and the study’s quality. Using small sample sizes can also distort the data, hurting the cross-sectional research‘s trustworthiness.
Wrong data analysis can lead to wrong conclusions, hurting the research’s credibility. Other issues include ignoring ethics and not focusing on real community problems. It’s important to pick topics that matter to our communities. Reading widely and getting advice from mentors can help us find good topics, like those listed at this link.
Effective Strategies to Overcome Challenges
To beat these challenges, we need good strategies. First, we must clearly define our research questions. Using strict sampling methods makes the data more reliable. Doing detailed reviews before analyzing the data helps us spot problems early.
Staying current with new methods and ethical rules is also key. This way, we can handle the complex issues of cross-sectional research well in 2024 and later. Knowing the best ways to do research and understanding the challenges helps us make valuable contributions to this field.
Response Bias Mitigation Techniques
Response bias is a big issue in cross-sectional research. To make our results reliable, we use mitigation techniques. Ensuring respondents’ anonymity and confidentiality helps them be honest. It’s key to tackle biases like social desirability and recall bias for valid data.
Using non-leading and neutral questions helps reduce response bias. By avoiding loaded language, we get more accurate answers. We aim for a diverse sample to make our research more representative.
Methodology matters a lot. Starting with focus groups can improve our questions and clear up confusion. Techniques like randomized response can also help with sensitive topics. Plus, sharing best practices with stakeholders helps everyone understand how to fight these biases effectively.
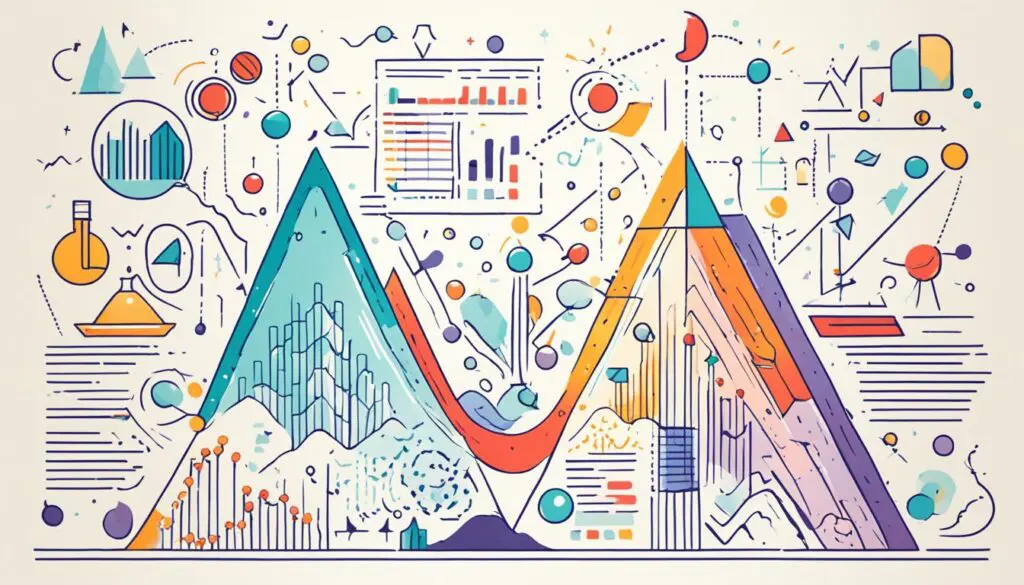
These strategies boost accuracy and trust in our research. With today’s issues like tech companies’ power and privacy concerns9, these techniques are crucial. The debate on data ethics highlights the need for strong bias mitigation10.
Establishing Validity and Reliability Measures
In cross-sectional research, making sure our studies are valid and reliable is key. Validity means our research really measures what we want to study. This is very important, especially when looking at how events like COVID-19 affect people. Reliability means our measurements are consistent, which makes our findings trustworthy.
To get these right, we use several strategies. For validity, we pick well-known measurement scales. Using triangulating data from different sources gives us a fuller picture. And, testing our surveys first helps us make sure they work well with our audience.
Knowing about the different levels of measurement helps improve our validity. Using various reliability methods, like checking consistency, keeps our research quality high. For example, choosing 100 students from a larger group with different backgrounds makes our data more representative11.
It’s also important to avoid common mistakes in research. Bad research questions can hurt both validity and reliability. Things like not doing good literature reviews or collecting data poorly can also mess up our results12. Knowing these issues helps us do better research.
Finally, as we think about how things might change after the pandemic, keeping our research valid and reliable is crucial. It’s our job to keep up with changes and make sure our research stays strong against false information and new public views2.
Cross-sectional vs. Longitudinal Studies
Exploring the differences between Cross-sectional Studies and Longitudinal Studies shows each has its own role. Cross-sectional Studies give us a snapshot of data at one point in time. They help us understand how things are related right now. On the other hand, Longitudinal Studies follow the same people over time. They help us see how things change and what causes them to change.
The design of Cross-sectional Studies is to gather lots of information all at once. This lets researchers find out what might cause things and what effects they have13. They are quick and don’t cost much, which is good for researchers with tight budgets14. But, Longitudinal Studies can show how things change over time. They give us deep insights into how behaviors or health conditions change15.
Both types of studies have their pros and cons. Cross-sectional Studies don’t tell us about how often diseases start (incidence) and aren’t great for proving cause and effect14. Longitudinal Studies take more time and money but give us detailed insights into health trends and behaviors over time15. Picking the right study type depends on what we want to learn and what resources we have.
Conclusion
Summary: Cross-sectional research is key to understanding our complex world. It helps us see how different groups and events interact. But, we must be careful to avoid mistakes that could change our results. We need to make sure our data collection is clear and our samples are well-chosen1612.
Looking ahead to 2024–2025, we plan to use the best methods to tackle tough issues. By improving our methods and sticking to scientific standards, we can get better insights from our studies. It’s important to keep learning about new research methods to make our work more useful17.
Today, we face big challenges that need reliable data from cross-sectional research. By staying flexible and meeting new needs, we can make our research count. To get better at writing research papers, we can check out this guide on writing rebuttal letters.
FAQ
What is cross-sectional research?
What are the key advantages of conducting cross-sectional studies?
Why is sample size determination important in cross-sectional research?
What data collection methods are typically used in cross-sectional research?
How can we minimize response bias in our studies?
What measures can be taken to ensure validity and reliability in our research findings?
What are the main differences between cross-sectional and longitudinal studies?
Source Links
- https://publichealth.jhu.edu/academics/academic-program-finder/training-grants/mixed-methods-research-training-program-for-the-health-sciences/about-the-program/why-mixed-methods
- https://www.pewresearch.org/internet/2021/02/18/experts-say-the-new-normal-in-2025-will-be-far-more-tech-driven-presenting-more-big-challenges/
- https://environment.yale.edu/courses/
- https://www.mdpi.com/2076-3417/13/12/7082
- https://my.cgu.edu/transdisciplinary/fellowships/research-award/
- https://resources.environment.yale.edu/courses
- https://agb.org/blog-post/whats-your-viewpoint-survey/
- https://pt.slideshare.net/chocolatelover02/survey-design-1
- https://www.pewresearch.org/internet/2021/02/18/worries-about-life-in-2025/
- https://asean.org/wp-content/uploads/2024/02/ASEAN-Guide-on-AI-Governance-and-Ethics_beautified_201223_v2.pdf
- https://www.slideshare.net/slideshow/mph5075-1ppt/255832312
- https://www.linkedin.com/advice/0/what-most-common-mistakes-when-designing-research
- https://www.slideshare.net/slideshow/study-session-14pptx/251438171
- https://es.slideshare.net/PreethiSelvaraj2/cross-sectional-study/5
- https://editverse.com/longitudinal-data-analysis-in-epidemiology-explained-2/
- https://www.law.uga.edu/student-handbook
- https://www.crisisgroup.org/global/sb11-ten-challenges-un-2023-2024