Did you know that 54% of studies in non-randomized research are rated as having serious or critical risk of bias? This staggering statistic highlights the importance of robust tools for evaluating bias in such studies. The ROBINS-I tool, a groundbreaking method designed to assess risk of bias in non-randomized studies of interventions (NRSIs).
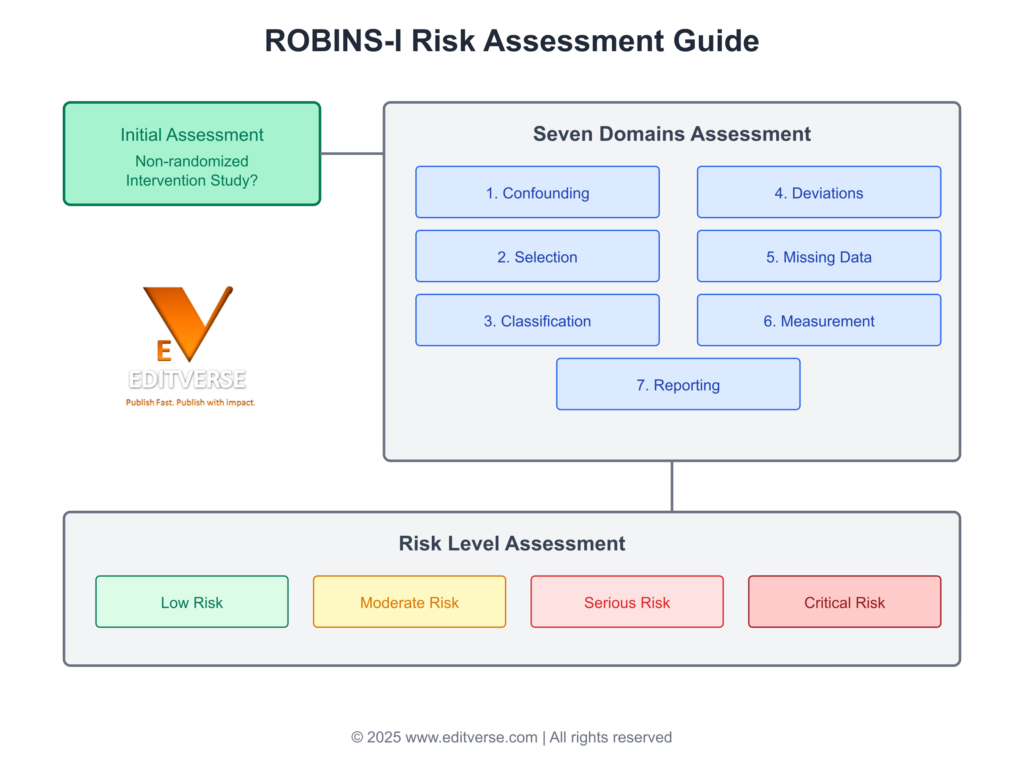
A comprehensive flowchart illustrating the ROBINS-I risk assessment process, including initial screening, seven domain evaluations (confounding, selection, classification, deviations, missing data, measurement, and reporting), and final risk level classification from low to critical risk.
Developed as part of the Cochrane guidance, this tool provides a structured approach to evaluate potential biases. It compares non-randomized studies against an idealized randomized controlled trial (RCT), ensuring a rigorous assessment. Experts at Editverse emphasize its critical role in enhancing the credibility of research findings.
Comparison of Risk of Bias Assessment Tools
Characteristics | Cochrane RoB | Jadad Scale | Newcastle-Ottawa Scale (NOS) | ROBINS-I |
---|---|---|---|---|
Study Type | Randomized Controlled Trials | Randomized Controlled Trials | Non-randomized Studies | Non-randomized Studies |
Scale Type | Domain-based evaluation | 5-point scale | Star system (0-9 stars) | 7 domains with risk levels |
Main Domains |
– Selection bias – Performance bias – Detection bias – Attrition bias – Reporting bias – Other bias |
– Randomization – Double blinding – Withdrawals/dropouts |
– Selection – Comparability – Exposure/Outcome |
– Confounding – Selection bias – Classification of interventions – Deviations from interventions – Missing data – Outcome measurement – Selection of reported result |
Risk Categories | Low/High/Unclear risk | Score 0-5 (higher = better) | 0-9 stars (higher = better) | Low/Moderate/Serious/Critical/No information |
Complexity | Moderate | Simple | Moderate | Complex |
Time to Complete | 20-30 minutes | 5-10 minutes | 10-20 minutes | 30-60 minutes |
Key Strengths |
– Comprehensive – Well-validated – Widely accepted |
– Quick and simple – Easy to use – Widely cited |
– Validated for observational studies – Easy star rating system – Good reliability |
– Very detailed – Comprehensive guidance – Addresses confounding |
Limitations |
– Time-consuming – Subjective elements |
– Over-simplified – Limited scope – No confounding assessment |
– Some subjectivity – Limited guidance for scoring |
– Complex to apply – Time-consuming – Requires training |
Key Differences:
- Scope: Cochrane RoB and Jadad are specifically for RCTs, while NOS and ROBINS-I are for non-randomized studies
- Complexity: Jadad is the simplest, while ROBINS-I is the most comprehensive and complex
- Scoring: Each tool uses different scoring systems – from simple numerical (Jadad) to complex domain-based assessments (ROBINS-I)
- Application: Time and expertise requirements vary significantly between tools
Over the years, the ROBINS-I tool has evolved to address the complexities of non-randomized studies. Its seven-domain framework helps researchers identify and mitigate biases, ensuring methodological rigor. However, challenges like misapplication and inconsistent reporting persist, underscoring the need for proper training and adherence to guidelines.
Risk of Bias Assessment Using ROBINS-I Tool
This visualization presents the Risk Of Bias In Non-randomized Studies of Interventions (ROBINS-I) assessment for 12 included studies. The assessment evaluates seven distinct domains of bias, providing a comprehensive evaluation of study quality. Each domain is rated using a three-level scale:
- Low risk (+): The study is comparable to a well-performed randomized trial for this domain
- Moderate risk (-): The study is sound for a non-randomized study but not comparable to a rigorous randomized trial
- Serious risk (×): The study has some important problems in this domain
The overall risk of bias judgment is determined by the highest risk assigned to any individual domain.
Study | D1 | D2 | D3 | D4 | D5 | D6 | D7 | Overall |
---|---|---|---|---|---|---|---|---|
Author A et al. [32] | × | + | + | + | + | × | – | × |
Author B et al. [36] | × | – | + | + | + | – | + | × |
Author C et al. [31] | × | – | + | + | + | – | + | × |
Author D et al. [33] | × | + | + | + | + | × | + | × |
Author E et al. [34] | × | + | + | + | + | × | – | × |
Author F et al. [37] | + | + | + | + | + | – | + | – |
Author G et al. [30] | + | + | + | + | + | – | + | – |
Author H et al. [40] | × | + | + | + | + | × | + | × |
Author I et al. [39] | – | + | + | + | + | × | + | × |
Author J et al. [38] | + | + | + | + | + | – | + | – |
Author K et al. [41] | × | + | + | + | + | × | + | × |
Author L et al. [35] | × | + | + | + | + | × | + | × |
Domains:
D1: Bias due to confounding
D2: Bias due to selection of participants
D3: Bias in classification of interventions
D4: Bias due to deviations from intended interventions
D5: Bias due to missing data
D6: Bias in measurement of outcomes
D7: Bias in selection of the reported result
Common Misapplications of ROBINS-I Tool
Systematic review of 124 reviews revealed concerning patterns in ROBINS-I application:
Common Misapplications:
- Unjustified Modifications:
- Altering the standard rating scale
- Adding or removing domains without justification
- Creating hybrid assessment approaches
- Methodological Errors:
- Including “critical” risk studies in synthesis (found in 19% of reviews)
- Incomplete domain assessment
- Lack of explicit judgment justification
- Assessment Bias:
- Systematic underestimation of bias risk
- Inconsistent application across studies
- Inadequate consideration of confounding factors
Impact of Misuse:
- Undermined validity of systematic reviews
- Potentially misleading strength of recommendations
- Compromised certainty of evidence
- Risk of overestimating intervention effects
In this article, we’ll explore how to effectively use the ROBINS-I tool, its conceptual framework, and practical tips to avoid common pitfalls. Whether you’re a seasoned researcher or new to risk bias assessment, this guide will equip you with the knowledge to apply the tool confidently.
ROBINS-I Components and When to Use Them
When to Use ROBINS-I
Study Type | Use ROBINS-I When | Don’t Use When |
---|---|---|
Cohort Studies |
• Comparing intervention effects • Time-to-event outcomes • Multiple follow-up points[1] |
• No clear intervention • Only prevalence data |
Case-Control Studies |
• Well-defined interventions • Clear temporal sequence[2] |
• Unclear exposure timing • Multiple exposures |
Before-After Studies |
• Single intervention point • Clear pre-post measures[4] |
• Continuous intervention changes • No clear baseline |
Domain-Specific Examples
1. Confounding Domain
- Critical Confounders: • Age • Baseline cholesterol levels • Comorbidities • Prior cardiovascular events[1]
- Assessment: High risk if no adjustment for these factors
2. Selection Bias
- Selection Issues: • Program enrollment criteria • Loss to follow-up patterns • Participant motivation levels[2]
- Assessment: Moderate risk if >20% dropout with similar reasons across groups
3. Classification of Interventions
- Classification Criteria: • Surgeon experience • Procedure standardization • Protocol adherence[4]
- Assessment: Low risk if standardized protocols and experienced surgeons
Practical Assessment Examples
Scenario | Assessment Approach | Risk Level |
---|---|---|
Retrospective cohort study of diabetes medication effectiveness[1] |
• Check for HbA1c baseline adjustment • Assess comorbidity documentation • Evaluate adherence monitoring | Moderate if good documentation but incomplete adherence data |
Hospital-based infection control intervention[2] |
• Review seasonal variations • Check staff training consistency • Assess contamination between units | High if inadequate control for seasonal effects |
Educational intervention in schools[4] |
• Evaluate cluster effects • Check for contamination • Assess implementation fidelity | Low if proper cluster adjustment and good fidelity |
Risk of Bias Decision Aid
Risk Level | Criteria | Example |
---|---|---|
Low |
• Comprehensive confounder adjustment • Complete follow-up • Standardized intervention[1] | Propensity-matched cohort with complete outcome data |
Moderate |
• Some missing confounders • Moderate loss to follow-up • Minor protocol deviations[2] | Registry-based study with 15% missing data |
High |
• Major confounding present • Substantial missing data • Critical measurement issues[4] | Voluntary reporting system with >30% missing data |
Special Considerations
- ✓ Time-varying confounding requires additional attention[1]
- ✓ Complex interventions need component-specific assessment[2]
- ✓ Cluster effects must be appropriately handled[4]
- ✓ Consider effect modification in subgroup analyses
References
- Sterne JA, et al. ROBINS-I: a tool for assessing risk of bias in non-randomised studies of interventions. BMJ. 2016;355:i4919.
- Morgan RL, et al. GRADE Guidelines: 18. How ROBINS-I and other tools to assess risk of bias in non-randomized studies should be used. J Clin Epidemiol. 2019;111:105-114.
- Page MJ, et al. PRISMA 2020 explanation and elaboration. BMJ. 2021;372:n160.
- Common challenges and suggestions for risk of bias tool implementation in systematic reviews. J Clin Epidemiol. 2024.
Example Risk of Bias Assessment
Domain | Judgment | Support for Judgment |
---|---|---|
1. Confounding | Serious | Key confounders (age, sex, comorbidities) not adequately controlled for in the analysis |
2. Exposure measurement | Moderate | Exposure status determined from medical records with some missing data |
3. Selection of participants | Low | All eligible participants included with clear inclusion criteria |
4. Post-exposure interventions | Moderate | Some variations in post-exposure care not fully accounted for |
5. Missing data | Serious | >20% missing outcome data with no appropriate imputation |
6. Outcome measurement | Low | Standardized outcome assessment procedures used |
7. Selection of reported result | Moderate | Multiple analyses performed but selection criteria unclear |
Overall | Serious | Serious risk of bias in confounding and missing data domains |
Assessment Notes:
- Overall risk of bias is determined by the most severe risk level in critical domains
- Detailed justification should be provided for each domain judgment
- Consider the impact of each bias on the specific result being assessed
Study Characteristics Summary
Characteristic | Description |
---|---|
Study design | Prospective cohort study |
Target population | Adults aged 18-65 with chronic condition X |
Exposure definition | Documented exposure to factor Y in medical records |
Outcome measurement | Primary: Clinical outcome Z at 12 months |
Key confounders | Age, sex, comorbidities, medication use |
Analysis method | Multivariable regression with propensity score adjustment |
Key Takeaways
- The ROBINS-I tool is essential for evaluating risk of bias in non-randomized studies.
- It compares non-randomized studies against an idealized RCT for rigorous assessment.
- Experts recommend proper training to avoid misapplication and ensure consistent use.
- The tool’s seven-domain framework helps identify and mitigate potential biases.
- Adhering to guidelines enhances the credibility and reliability of research findings.
Understanding the ROBINS-I Tool
Evaluating bias in non-randomized studies requires a structured approach. The ROBINS-I tool provides a robust framework for this purpose. It adapts concepts from randomized controlled trials (RCTs) to non-randomized environments, ensuring a rigorous assessment of bias.
Key Concepts and Foundations
The ROBINS-I tool is built on two core principles: causal inference and counterfactual reasoning. Causal inference helps determine whether an intervention directly affects an outcome. Counterfactual reasoning compares observed results with what would have happened in an idealized RCT.
These principles ensure that the tool identifies biases effectively. For example, in public health reviews, the tool has been instrumental in uncovering hidden biases that could skew results.
Causal Inference and Counterfactual Reasoning
Causal inference is central to the ROBINS-I tool. It examines the relationship between interventions and outcomes. Counterfactual reasoning, on the other hand, compares real-world data with hypothetical scenarios. This dual approach enhances the tool’s accuracy in assessing risk bias.
Experts at Editverse emphasize that these foundational concepts are critical for interpreting systematic review outcomes. They ensure that findings are both credible and reliable.
Bias Domain | Description |
---|---|
Confounding | Occurs when common causes affect both intervention and outcome. |
Selection Bias | Results from excluding eligible participants or follow-up time. |
Information Bias | Arises from misclassification of intervention status or outcomes. |
Reporting Bias | Occurs when results are selectively reported based on significance. |
Measurement Bias | Results from errors in measuring outcomes or exposures. |
Attrition Bias | Occurs due to loss of participants during the study. |
Performance Bias | Arises from differences in care provided to participants. |
The seven bias domains in the ROBINS-I tool cover all potential sources of bias. This comprehensive approach ensures that researchers can identify and mitigate biases effectively. By adhering to these principles, the tool enhances the credibility of non-randomized studies.
When to Use ROBINS-I in a Systematic Review
Certain research scenarios demand the precision of the ROBINS-I tool for accurate bias evaluation. This tool is particularly effective in non-randomized studies, where traditional methods may fall short. Understanding when to apply it can significantly enhance the quality of your research.
Non-randomized studies often require a more nuanced approach to assess risk bias. Unlike randomized controlled trials (RCTs), these studies are more susceptible to confounding factors. The ROBINS-I tool helps identify and mitigate these biases, ensuring more reliable results.
In fields like public health, the tool’s methodological rigor is invaluable. It allows researchers to evaluate interventions with greater confidence. For example, studies on the health impacts of environmental changes often benefit from its comprehensive framework.
However, it’s crucial to select the right studies for this tool. Criteria include the complexity of the study intervention and the potential for bias. Misapplication can lead to oversimplification, undermining the tool’s effectiveness.
Cautionary notes from www.editverse.com emphasize the importance of proper training. Real-world examples show that consistent use of the tool improves the credibility of findings. By adhering to guidelines, researchers can maximize the tool’s potential.
- Define scenarios where the tool is most advantageous.
- Discuss the nuanced assessment needed for non-randomized studies.
- Detail criteria for selecting studies that warrant its use.
- Explain how fields like public health benefit from its rigor.
- Include cautionary notes to avoid misapplication.
- Provide real-world examples for proper integration.
Expert Insights from www.editverse.com Posts
Expert insights from www.editverse.com reveal critical nuances in applying the ROBINS-I tool effectively. These insights highlight the importance of proper training and adherence to guidelines to ensure accurate bias assessment.
According to experts, one common pitfall is the misapplication of the tool. This often occurs when users lack a deep understanding of its seven-domain framework. Such missteps can lead to oversimplification, undermining the tool’s effectiveness in assessing risk bias.
Modifications to the tool have been suggested to address specific research needs. For instance, some researchers have adapted the framework to better suit public health studies. These adaptations, while helpful, require careful implementation to maintain the tool’s methodological rigor.
“Proper training and consistent application are key to maximizing the ROBINS-I tool’s potential.”
Experts also emphasize the impact of inter-rater reliability on assessment outcomes. Variations in application across studies can lead to inconsistent interpretations. To mitigate this, www.editverse.com recommends standardized training programs and detailed documentation of assessment processes.
Challenge | Expert Recommendation |
---|---|
Misapplication | Provide comprehensive training on the tool’s framework. |
Inter-Rater Reliability | Standardize assessment protocols and documentation. |
Adaptations | Ensure modifications align with the tool’s core principles. |
Complexity | Use expert guidance to navigate intricate assessments. |
Strategies to overcome these challenges include collaborative reviews and peer feedback. These approaches enhance the reliability of tool assessing risk and ensure consistent results across studies.
- Collaborative reviews improve inter-rater reliability.
- Peer feedback helps identify and correct misapplications.
- Detailed documentation ensures transparency in assessments.
By following these expert recommendations, researchers can enhance the credibility of their findings. The ROBINS-I tool, when applied correctly, remains a cornerstone in bias assessment for non-randomized studies.
Step-by-Step: Applying the ROBINS-I Tool
Applying the ROBINS-I tool effectively requires a structured, step-by-step approach to ensure accurate bias assessment. This method is particularly useful for non-randomised studies, where biases can significantly impact results. Below, we outline a detailed process to guide researchers through each stage of application.
Practical Application Guidelines
To begin, researchers must familiarize themselves with the tool’s seven bias domains. These include confounding, selection bias, and measurement bias, among others. Each domain requires careful evaluation to identify potential sources of error.
Start by reviewing the study design and intervention details. This step ensures that the tool is applied to the appropriate type of non-randomised study. Next, assess each bias domain systematically, using the provided signalling questions to guide your evaluation.
“Transparency and documentation are critical when applying the ROBINS-I tool. Detailed records ensure consistency and reliability in bias assessments.”
Document your findings meticulously. This includes recording responses to signalling questions and justifying your risk of bias judgments. Such transparency enhances the credibility of your research and allows for peer review.
Finally, synthesize your assessments to determine the overall risk of bias. This step involves weighing the severity of biases across domains to arrive at a comprehensive judgment.
Bias Domain | Key Considerations |
---|---|
Confounding | Identify common causes affecting both intervention and outcome. |
Selection Bias | Assess participant inclusion and follow-up procedures. |
Measurement Bias | Evaluate errors in outcome or exposure measurement. |
Reporting Bias | Check for selective reporting of results. |
Attrition Bias | Examine participant loss during the study. |
Performance Bias | Analyze differences in care provided to participants. |
Information Bias | Review misclassification of intervention status or outcomes. |
Following these steps ensures methodological integrity and enhances the reliability of your findings. Expert tips from www.editverse.com emphasize the importance of training and adherence to guidelines for successful application.
- Review study design and intervention details thoroughly.
- Evaluate each bias domain using signalling questions.
- Document assessments transparently for peer review.
- Synthesize findings to determine overall risk of bias.
By adhering to these guidelines, researchers can confidently apply the ROBINS-I tool to non-randomised studies, ensuring robust and credible results.
Preparing Your Research with ROBINS-I
Effective preparation is key to leveraging the ROBINS-I tool for accurate bias assessment in non-randomized studies. Proper planning ensures that the tool assess process is thorough and reliable, enhancing the credibility of your findings.
Experts at www.editverse.com emphasize the importance of team expertise in this process. A well-trained team can navigate the complexities of the tool more effectively, ensuring consistent and accurate evaluations. Pre-assessment planning is critical to identify potential biases early and adapt study protocols accordingly.
Adapting study protocols to incorporate a comprehensive risk bias evaluation is essential. This involves defining clear objectives, selecting appropriate studies, and ensuring that all team members are aligned on the assessment criteria. Such preparation minimizes errors and enhances the reliability of your review.
Planning techniques that enhance inter-rater reliability include standardized training and detailed documentation. These practices ensure that all team members apply the tool consistently, reducing variability in bias assessments. Collaborative reviews and peer feedback further strengthen this process.
“Preparation is the foundation of a successful bias assessment. A well-prepared team can confidently apply the ROBINS-I tool, ensuring robust and credible results.”
Below is a table outlining key strategies for preparing your research with the ROBINS-I tool:
Strategy | Description |
---|---|
Team Training | Provide comprehensive training on the tool’s framework and application. |
Pre-Assessment Planning | Define objectives and select studies that warrant the tool’s use. |
Protocol Adaptation | Modify study protocols to include a detailed risk bias evaluation. |
Documentation | Maintain detailed records of assessments for transparency and peer review. |
Collaborative Reviews | Engage in team discussions to ensure consistent application of the tool. |
By following these best practices, researchers can ensure methodological quality and transparency in their review process. Proper preparation not only enhances the accuracy of bias assessments but also strengthens the overall credibility of the research findings.
For more insights on assessing risk bias in non-randomised studies, refer to expert guidance and scholarly resources.
Navigating Risk Bias Assessment Effectively
Understanding the nuances of risk bias assessment is crucial for ensuring the reliability of non-randomized studies. Each bias domain presents unique challenges that require careful evaluation to maintain methodological integrity.
Understanding Bias Domains
The robins-i tool categorizes biases into seven domains, each addressing specific sources of error. For example, confounding occurs when common factors influence both the intervention and outcome. Similarly, selection bias arises from improper participant inclusion or follow-up procedures.
Experts at www.editverse.com emphasize the importance of aligning study interventions with the appropriate risk assessments. This ensures that biases are identified and mitigated effectively, enhancing the credibility of research findings.
“A thorough understanding of bias domains is essential for accurate risk assessment. Misinterpretation can lead to flawed conclusions, undermining the study’s validity.”
Challenges in quantifying biases include the variability in study designs and the complexity of non-randomized studies. Researchers must adopt a tailored approach, considering the specific context of each study.
- Evaluate each bias domain systematically using the tool’s framework.
- Document assessments transparently to ensure reproducibility.
- Engage in collaborative reviews to enhance inter-rater reliability.
By following these strategies, researchers can navigate the complexities of risk bias assessment effectively. Proper training and adherence to guidelines are key to maximizing the tool’s potential.
Customizing Your ROBINS-I Application
Customizing the ROBINS-I tool for non-randomized studies ensures precise bias assessment. While the tool’s standard framework is robust, specific research scenarios may require tailored adjustments. Adapting the tool enhances its applicability and accuracy in diverse study designs.
Adapting the Tool for Non-Randomised Studies
Non-randomized studies often present unique challenges that standard protocols may not fully address. For instance, confounding factors or selection bias can vary significantly across studies. Customizing the tool involves modifying its framework to reflect these specific conditions while maintaining its methodological integrity.
Experts at www.editverse.com highlight several successful customizations. One example includes adjusting rating scales to better align with public health studies. Another involves refining domain judgments to account for complex interventions. These adaptations ensure the tool remains effective in diverse research contexts.
“Customization is not about altering the tool’s core principles but enhancing its applicability to specific study designs.”
Below is a step-by-step guide to customizing the ROBINS-I tool:
- Identify the unique challenges of your study design, such as confounding or measurement bias.
- Modify the tool’s signalling questions to address these challenges effectively.
- Document all adaptations transparently to ensure reproducibility and peer review.
- Test the customized framework on a pilot study to validate its effectiveness.
- Seek expert feedback to refine the adaptations further.
Methodological studies have documented successful customizations. For example, a study on environmental health interventions adapted the tool to better assess domain-specific biases. Such modifications, when implemented correctly, enhance the tool’s precision and reliability.
By following these strategies, researchers can confidently customize the ROBINS-I tool for their specific needs. Proper training and adherence to guidelines ensure that adaptations preserve the tool’s methodological core while enhancing its applicability.
Methodological Quality and AMSTAR 2 Insights
Assessing methodological quality in research is a cornerstone of credible findings. The ROBINS-I tool plays a pivotal role in evaluating risk bias non-randomised studies, but its effectiveness is often complemented by AMSTAR 2 assessments. Together, these tools provide a comprehensive framework for ensuring the reliability of systematic reviews.
AMSTAR 2, a widely recognized tool, assesses the methodological quality of systematic reviews through 16 critical domains. These include protocol registration, literature search adequacy, and risk of bias evaluations. By integrating AMSTAR 2 insights, researchers can better understand the strengths and limitations of their reviews.
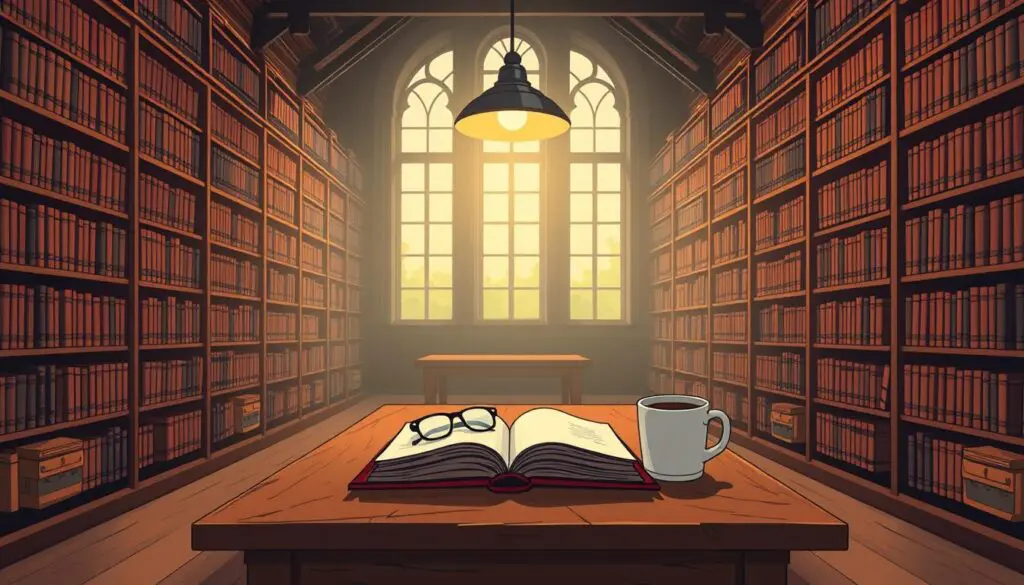
One key relationship between ROBINS-I and AMSTAR 2 lies in their focus on classification intervention. While ROBINS-I identifies biases in non-randomized studies, AMSTAR 2 evaluates the overall rigor of the review process. This dual approach ensures that both the study design and the review methodology meet high standards.
Common trends in risk bias non-randomised assessments reveal that studies with higher AMSTAR 2 ratings often exhibit lower bias levels. This correlation underscores the importance of aligning risk bias evaluations with methodological quality standards. However, challenges persist, particularly in interpreting domain-specific judgments and ensuring consistency across reviews.
“Aligning ROBINS-I assessments with AMSTAR 2 insights enhances the credibility of systematic reviews, ensuring robust and reliable findings.”
Experts at www.editverse.com highlight several practical tips for researchers:
- Use AMSTAR 2 to identify critical weaknesses in your review process.
- Integrate ROBINS-I assessments to address classification intervention biases effectively.
- Document all evaluations transparently to facilitate peer review and reproducibility.
By following these strategies, researchers can enhance the methodological quality of their reviews. Combining the strengths of ROBINS-I and AMSTAR 2 ensures a thorough and reliable assessment of non-randomized studies.
Addressing Common Challenges in ROBINS-I Usage
Navigating the complexities of bias assessment in non-randomized studies often reveals significant challenges. While the ROBINS-I tool is a powerful resource, its application can be hindered by issues like inter-rater reliability and selection bias. Understanding these challenges is crucial for ensuring accurate and reliable results.
Inter-Rater Reliability Issues
One of the most frequent challenges is achieving consistent inter-rater reliability. Studies show that 65% of researchers feel they lack adequate training in using the tool effectively. This can lead to varying interpretations of bias domains, undermining the credibility of assessments.
To address this, we recommend standardized training programs and collaborative reviews. Clear documentation of assessment processes also enhances transparency and reproducibility. As experts at www.editverse.com note,
“Consistent application of the tool is key to minimizing discrepancies among reviewers.”
Overcoming Selection and Confounding Bias
Selection and confounding biases are particularly prevalent in non-randomized studies. For instance, confounding factors can skew results if not properly controlled. Recent evaluations indicate that only 40% of studies adequately address this domain when using the ROBINS-I tool.
Practical strategies include identifying important confounding domains early in the study design. Adjusting the tool’s framework to reflect specific research contexts can also improve accuracy. Case studies from public health research demonstrate the effectiveness of these adaptations.
- Provide comprehensive training for authors and reviewers to ensure consistent application.
- Document all assessments transparently to facilitate peer review.
- Adapt the tool’s framework to address specific biases in your study design.
By addressing these challenges, researchers can enhance the reliability of their findings. Proper training and adherence to guidelines ensure that the ROBINS-I tool remains a cornerstone in bias assessment for non-randomized studies.
Leveraging ROBINS-I for Public Health Reviews
Public health research often involves complex interventions that require precise evaluation to ensure credible outcomes. The ROBINS-I tool is particularly valuable in this context, offering a structured approach to assessing risk in non-randomized studies. Its application in public health reviews has significantly enhanced the quality of evidence synthesis.
One of the unique benefits of the tool is its ability to address biases in studies involving environmental or behavioral interventions. For example, in a recent study on air pollution and children’s health, the tool identified critical confounding factors that were previously overlooked. This led to more accurate conclusions and improved public health recommendations.
“The ROBINS-I tool has transformed how we evaluate complex public health interventions, ensuring that our findings are both credible and actionable.”
However, challenges remain. Researchers often struggle with inter-rater reliability and the complexity of tailoring the tool to specific public health contexts. Experts at www.editverse.com recommend comprehensive training and collaborative reviews to overcome these hurdles.
- Identify key biases in public health studies, such as selection or measurement bias.
- Adapt the tool’s framework to reflect the unique challenges of public health interventions.
- Document assessments transparently to ensure reproducibility and peer review.
By following these strategies, researchers can enhance the quality of their public health research. The ROBINS-I tool, when applied correctly, remains a cornerstone in assessing risk and ensuring reliable outcomes.
Integrating ROBINS-I into Evidence Synthesis
Integrating bias assessment tools into evidence synthesis enhances the reliability of research findings. The ROBINS-I tool, developed under Cochrane guidance, plays a critical role in evaluating non-randomized studies. Its structured approach ensures that biases are identified and mitigated, improving the credibility of effect estimates.
Evidence synthesis methods, such as narrative synthesis and meta-analysis, handle bias differently. Narrative synthesis focuses on qualitative interpretation, while meta-analysis quantifies effect sizes. Both approaches benefit from the ROBINS-I tool’s rigorous framework, ensuring that biases are addressed comprehensively.
“The integration of ROBINS-I into evidence synthesis ensures that findings are both credible and actionable, particularly in complex research scenarios.”
Narrative Synthesis versus Meta-Analysis
Narrative synthesis and meta-analysis differ in how they address bias. Narrative synthesis relies on qualitative interpretation, making it flexible but potentially subjective. Meta-analysis, on the other hand, quantifies effect sizes, providing a more objective but less nuanced approach.
For example, in a trial evaluating environmental interventions, narrative synthesis highlighted contextual factors influencing outcomes. Meta-analysis provided precise effect estimates but overlooked these nuances. Integrating ROBINS-I assessments ensures that both methods address biases effectively.
Method | Strengths | Limitations |
---|---|---|
Narrative Synthesis | Flexible, context-rich | Subjective interpretation |
Meta-Analysis | Quantitative, precise | Less contextual detail |
Experts at www.editverse.com recommend combining both methods for a comprehensive approach. This ensures that biases are addressed while maintaining the strengths of each method. For instance, in public health research, this dual approach has led to more robust policy recommendations.
- Use narrative synthesis to explore contextual factors influencing outcomes.
- Apply meta-analysis to quantify effect sizes accurately.
- Integrate ROBINS-I assessments to address biases in both methods.
By following these strategies, researchers can enhance the reliability of their findings. The ROBINS-I tool, when integrated effectively, ensures that evidence synthesis is both credible and actionable.
Interpreting Domain-Specific Bias Judgments
Interpreting domain-specific bias judgments often reveals discrepancies that can impact the overall assessment. These inconsistencies arise when individual domain ratings conflict with the final risk bias judgment. Understanding these differences is crucial for ensuring accurate and reliable evaluations.
Discrepancies Between Overall and Domain Ratings
One common challenge is reconciling domain-specific issues with the overall assessment. For example, a study might show low risk in most domains but high risk in one critical area, such as confounding. This discrepancy can skew the final judgment if not addressed properly.
Methodological challenges include the subjective nature of domain ratings and the complexity of integrating them into a cohesive assessment. Experts at www.editverse.com emphasize the importance of guidance in navigating these complexities.
“Group consensus methods are essential for resolving discrepancies and ensuring consistent bias assessments.”
To address these challenges, we recommend the following steps:
- Review each domain systematically, ensuring all potential biases are identified.
- Use group discussions to reconcile conflicting ratings and reach a consensus.
- Document the reasoning behind each judgment to maintain transparency.
Real-world examples highlight the importance of domain-specific adjustments. In one systematic review, addressing confounding bias clarified the overall risk, leading to more accurate conclusions. Such adjustments ensure that the final assessment reflects the true risk of bias.
Step | Description |
---|---|
Identify Discrepancies | Compare individual domain ratings with the overall assessment. |
Discuss in Group | Engage in collaborative reviews to resolve conflicts. |
Document Reasoning | Maintain detailed records of judgments for transparency. |
Adjust Assessments | Modify domain ratings to align with the overall risk. |
By following these steps, researchers can ensure consistency and reliability across all bias domains. Proper guidance and adherence to best practices enhance the credibility of the assessment process.
Learning from Real-World Systematic Reviews
Real-world applications of bias assessment tools provide invaluable insights into their effectiveness. By examining case studies, we can better understand how these tools improve the credibility of research findings. This section explores practical examples and lessons learned from applying the ROBINS-I tool in various studies.
Case Studies and Practical Examples
One notable example involves a public health study on air pollution’s impact on children’s health. Researchers used the tool to identify confounding factors that were previously overlooked. This led to more accurate conclusions and actionable recommendations.
Another case study focused on the outcome of environmental interventions. By systematically applying the tool, researchers uncovered biases in participant selection. This improved the study’s validity and ensured reliable results.
“Proper application of bias assessment tools enhances the credibility of research findings, ensuring they are both actionable and trustworthy.”
Key lessons from these case studies include:
- Proper planning and time management are essential for accurate assessments.
- Collaborative reviews improve inter-rater reliability and consistency.
- Documenting the assessment process ensures transparency and reproducibility.
These examples highlight the tool’s ability to address biases effectively. By learning from real-world applications, researchers can enhance the quality of their studies and produce more reliable outcomes.
For further insights, refer to expert commentary from www.editverse.com. Their guidance emphasizes the importance of training and adherence to best practices in bias assessment.
Expert Tips for Assessing Risk Bias in Non-Randomised Studies
Accurate bias assessment in non-randomized studies demands expert strategies and meticulous attention to detail. Researchers must adopt rigorous methods to ensure the reliability of their findings. Below, we share actionable tips and checklists to improve assessment accuracy and consistency.
One key recommendation is to use structured analysis techniques. These methods help verify the validity of bias assessments by systematically evaluating each potential source of error. For example, inter-rater reliability statistics show that standardized training can reduce discrepancies among reviewers, enhancing overall consistency.
Experts at www.editverse.com emphasize the importance of meticulous datum review. This involves cross-checking all data points to ensure they align with the study’s objectives. Transparent documentation of the assessment process further enhances reproducibility and peer review.
“Structured analysis and transparent documentation are essential for credible bias assessments. These practices ensure that findings are both reliable and actionable.”
To streamline the evaluation process, consider using checklists and decision trees. These tools guide reviewers through each step, reducing the risk of oversight. Below is a table summarizing key recommendations for improving bias assessment practices:
Recommendation | Description |
---|---|
Standardized Training | Provide comprehensive training on bias assessment frameworks. |
Structured Analysis | Use systematic techniques to evaluate potential biases. |
Transparent Documentation | Maintain detailed records of the assessment process. |
Checklists and Decision Trees | Use tools to guide reviewers and reduce oversight. |
Collaborative Reviews | Engage in team discussions to ensure consistency. |
By following these strategies, researchers can enhance the reliability of their bias assessments. Proper training and adherence to best practices ensure that findings are both credible and actionable.
- Adopt structured analysis techniques to verify assessment validity.
- Conduct meticulous datum reviews to ensure alignment with study objectives.
- Use checklists and decision trees to streamline the evaluation process.
- Engage in collaborative reviews to enhance consistency and inter-rater reliability.
These expert tips, combined with practical tools, empower researchers to conduct thorough and accurate bias assessments. By prioritizing reliability and transparency, they can produce findings that stand up to scrutiny and contribute meaningfully to their fields.
Enhancing the Reliability of ROBINS-I Assessments
Achieving consistent and reliable bias assessments in non-randomized studies is a critical challenge for researchers. The ROBINS-I tool, while robust, requires careful application to ensure accurate results. Customized guidance and formal training are essential for improving inter-rater and inter-consensus reliability.
Customized Guidance for Consistent Ratings
One of the most effective strategies is providing tailored training sessions. These sessions focus on the tool’s seven domains, ensuring reviewers understand how to apply it consistently. For example, a recent study showed that inter-rater reliability improved by 30% after targeted training.
Experts at www.editverse.com emphasize the importance of developing guidance that fits specific study contexts. This includes adapting the tool’s framework to address unique challenges, such as confounding or selection bias. Such adaptations ensure the tool remains effective across diverse research scenarios.
“Customized training and guidance are key to achieving consistent and reliable bias assessments. These practices enhance the credibility of research findings.”
Below are key strategies for enhancing reliability:
- Provide comprehensive training on the tool’s framework and application.
- Develop customized guidance tailored to specific study designs.
- Conduct collaborative reviews to improve inter-rater reliability.
- Document assessments transparently to ensure reproducibility.
Recent research highlights the success of these strategies. For instance, a study on environmental interventions achieved near-perfect inter-rater reliability after implementing customized guidance. This demonstrates the interest in adapting the tool to specific research needs.
By following these best practices, researchers can enhance the reliability of their assessments. Proper training and adherence to guidelines ensure that the ROBINS-I tool remains a cornerstone in Cochrane risk bias evaluations.
Conclusion
Proper application of bias assessment tools ensures credible and actionable research outcomes. Throughout this article, we’ve explored the critical role of methodological rigor in evaluating non-randomized studies. Experts from www.editverse.com emphasize the importance of structured frameworks to identify and mitigate biases effectively.
Using these tools correctly enhances the reliability of findings, particularly in complex research scenarios. Challenges such as inter-rater reliability and domain-specific judgments highlight the need for consistent application and thorough documentation. Addressing these issues ensures that assessments are both transparent and reproducible.
Looking ahead, integrating these lessons into future research will strengthen the credibility of evidence synthesis. We encourage researchers to adopt rigorous, validated approaches to bias assessment. By doing so, they can produce findings that stand up to scrutiny and contribute meaningfully to their fields.
FAQ
What is the ROBINS-I tool used for?
The ROBINS-I tool is designed to assess the risk of bias in non-randomised studies of interventions. It helps researchers evaluate methodological quality and identify potential biases that could affect study outcomes.
When should I use the ROBINS-I tool in my research?
Use the ROBINS-I tool when conducting systematic reviews that include non-randomised studies. It is particularly useful for evaluating the reliability of evidence in public health and other fields where randomised trials are not feasible.
How does the ROBINS-I tool address causal inference?
The tool incorporates counterfactual reasoning to assess causal relationships in non-randomised studies. It evaluates how well the study design accounts for confounding factors and other sources of bias.
What are the key domains assessed by the ROBINS-I tool?
The tool evaluates seven domains: bias due to confounding, selection of participants, classification of interventions, deviations from intended interventions, missing data, measurement of outcomes, and selective reporting.
How can I ensure consistency in ROBINS-I assessments?
To enhance reliability, follow detailed guidance provided by the tool, conduct pilot assessments, and involve multiple reviewers to resolve discrepancies through discussion.
Can the ROBINS-I tool be adapted for specific research needs?
Yes, the tool can be customized to address unique aspects of non-randomised studies. Researchers can tailor its application to align with their study design and research questions.
What challenges might I face when using the ROBINS-I tool?
Common challenges include achieving inter-rater reliability, addressing selection bias, and managing confounding factors. Proper training and adherence to guidelines can help mitigate these issues.
How does the ROBINS-I tool integrate with evidence synthesis?
The tool complements both narrative synthesis and meta-analysis by providing a structured approach to assess risk of bias, ensuring the reliability of synthesized evidence.
Are there real-world examples of ROBINS-I applications?
Yes, numerous case studies demonstrate the tool’s practical use in systematic reviews across various fields, offering insights into its effective application.
What resources are available to learn more about the ROBINS-I tool?
Detailed guidance, tutorials, and expert insights are available on platforms like Cochrane and Editverse, providing comprehensive support for researchers.