Did you know that over 870 young adults and 610 adolescents joined the Human Connectome Project? This project aims to map the brain’s neural connections. It’s changing how we see cognition, neurological disorders, and the human mind’s potential.
Neuroscience and AI: Bridging the Gap Between Biological and Artificial Intelligence
The fields of neuroscience and artificial intelligence (AI) are increasingly intertwined, with each discipline informing and advancing the other. This synergy is leading to groundbreaking discoveries about the nature of intelligence and cognition, while also driving the development of more sophisticated AI systems.
Comparing Biological and Artificial Neural Networks
Understanding the similarities and differences between biological and artificial neural networks is crucial for advancing both fields:
Feature | Biological Neural Networks | Artificial Neural Networks |
---|---|---|
Basic Unit | Neuron | Artificial Neuron (Node) |
Connections | Synapses | Weighted Connections |
Learning Mechanism | Synaptic Plasticity | Backpropagation, Gradient Descent |
Processing | Parallel, Distributed | Often Sequential, Can be Parallel |
Energy Efficiency | Highly Efficient | Less Efficient, Improving |
Key Areas of Intersection
The intersection of neuroscience and AI is driving progress in several key areas:
- Neural Network Architectures: Insights from neuroscience are inspiring new AI architectures, such as spiking neural networks and neuromorphic computing systems[1].
- Learning Algorithms: Biological learning mechanisms like synaptic plasticity are informing the development of more efficient and adaptable machine learning algorithms[2].
- Cognitive Models: AI is being used to create and test computational models of cognitive processes, helping to validate and refine neuroscientific theories[3].
- Brain-Computer Interfaces: Advances in both fields are enabling the development of more sophisticated brain-computer interfaces for medical and technological applications[4].
Recent Breakthroughs
Several recent developments highlight the synergy between neuroscience and AI:
- The development of large language models like GPT-3, which have sparked discussions about the nature of language understanding and generation in both artificial and biological systems[5].
- Advancements in neuromorphic computing, creating chips that more closely mimic the structure and function of biological neural networks[6].
- The use of AI in decoding neural activity, leading to improved brain-computer interfaces and potential treatments for neurological disorders[7].
Ethical Considerations
As the fields of neuroscience and AI continue to converge, several ethical considerations emerge:
- Privacy concerns related to brain-computer interfaces and the potential for unauthorized access to neural data.
- The implications of creating artificial systems that closely mimic human cognitive processes.
- Ensuring equitable access to neurotechnology and AI-enhanced cognitive tools.
- The potential impact on human agency and decision-making as AI systems become more integrated with human cognition.
Future Directions
The continued collaboration between neuroscience and AI is likely to yield exciting developments in the coming years:
- More sophisticated brain-inspired AI architectures that can handle complex, real-world tasks with greater efficiency.
- Improved understanding of human cognition and consciousness through computational modeling and AI-assisted research.
- Advanced neuroprosthetics and brain-computer interfaces that can restore or enhance cognitive functions.
- AI systems that can adapt and learn more like biological systems, potentially leading to more general artificial intelligence.
Conclusion
The intersection of neuroscience and AI represents a frontier of scientific inquiry with immense potential for advancing our understanding of intelligence, both biological and artificial. As these fields continue to inform and inspire each other, we can expect groundbreaking discoveries that will shape the future of technology, medicine, and our understanding of the human mind.
References
[1] Pfeiffer, M., & Pfeil, T. (2018). Deep Learning With Spiking Neurons: Opportunities and Challenges. Frontiers in Neuroscience, 12, 774.
[2] Marblestone, A. H., Wayne, G., & Kording, K. P. (2016). Toward an Integration of Deep Learning and Neuroscience. Frontiers in Computational Neuroscience, 10, 94.
[3] Kriegeskorte, N., & Douglas, P. K. (2018). Cognitive computational neuroscience. Nature Neuroscience, 21(9), 1148-1160.
[4] Lebedev, M. A., & Nicolelis, M. A. L. (2017). Brain-Machine Interfaces: From Basic Science to Neuroprostheses and Neurorehabilitation. Physiological Reviews, 97(2), 767-837.
[5] Brown, T. B., et al. (2020). Language Models are Few-Shot Learners. arXiv:2005.14165.
[6] Davies, M., et al. (2018). Loihi: A Neuromorphic Manycore Processor with On-Chip Learning. IEEE Micro, 38(1), 82-99.
[7] Anumanchipalli, G. K., Chartier, J., & Chang, E. F. (2019). Speech synthesis from neural decoding of spoken sentences. Nature, 568(7753), 493-498.
The Human Connectome Project uses advanced neuroimaging techniques and computational modeling. It helps us understand the complex neural networks that control our thoughts, feelings, and actions. With artificial intelligence (AI) and machine learning, researchers are discovering new things about the brain.
This project could change neuroscience, psychiatry, and neurological disease treatment. By mixing neuroimaging data with computational models, scientists can learn more about the brain. This could lead to new treatments, early help, and brain-computer interfaces.
Key Takeaways
- The Human Connectome Project maps the brain’s neural connections with advanced tools and models.
- It uses AI and machine learning to understand brain function and neurological disorders.
- Its findings could change how we see the brain and help in treating diseases.
- It involves many young adults and teens, giving researchers a lot of data.
- By mixing brain scans with models, researchers can see how the brain works better.
Introduction to Computational Neuroimaging
Computational neuroimaging is a fast-growing field. It uses advanced imaging like functional MRI (fMRI), magnetoencephalography (MEG), and electroencephalography (EEG). These methods, combined with computational models, study how the brain works and affects behavior. Computational models in neuroimaging research offer deep insights into brain functions and behavior. They also provide tools to predict outcomes in clinical settings.
Computational Models in Neuroimaging Research
Computational models in neuroimaging research use what we already know to understand brain processes and behaviors. These models analyze neuroimaging data with machine learning (ML) algorithms. They use both supervised and unsupervised learning methods.
The Emergence of Computational Neuroimaging
The rise of computational neuroimaging comes from the growth of neuroimaging data and the need to make sense of it. Functional MRI (fMRI), magnetoencephalography (MEG), and electroencephalography (EEG) are key neuroimaging techniques used in studying the brain and mental health. Computational models are now key in these studies. They link data to brain mechanisms to understand brain-behavior links and predict outcomes.
“Computational models in neuroimaging research can provide mechanistic insights into the neural processes underlying cognition and behavior, as well as predictive tools for clinical outcomes.”
Strengths and Limitations of Computational Neuroimaging
Computational Neuroscience and advanced Neuroimaging have grown closer, showing both big benefits and challenges. A major plus of computational Neuroimaging is how it connects brain workings with thinking processes. This helps us understand the brain better. By mixing computational models with neuroimaging data, researchers learn more about the brain’s role in thinking and behavior.
Also, computational Neuroimaging could greatly improve how neuroimaging helps in healthcare. Machine learning can look at neuroimaging data to find patterns that might show certain brain or mental health issues. This could lead to better ways to diagnose and treat these conditions.
But, there are worries about how well lab studies match real-life thinking and actions. Some neuroimaging methods, like fMRI, might not catch all the details of brain activity. This could be because they’re not very good at showing the timing or location of brain activity.
- Computational models can bridge the gap between neural mechanisms and cognitive processes.
- Computational Neuroimaging enhances the predictive power of neuroimaging for clinical applications.
- Concerns about the ecological validity of lab-based Neuroimaging studies.
- Limited spatiotemporal resolution of some neuroimaging techniques.
Fixing these issues and making computational models work better with Neuroimaging data is a big challenge. By improving methods and trying new things, researchers can learn more about how the brain, thinking, and behavior are linked.
“The integration of computational models with neuroimaging data holds great promise, but we must be mindful of the limitations and work to enhance the ecological validity and spatiotemporal resolution of our research.”
Comparison with Alternative Neuroscience Methods
Neuroimaging is a key tool in studying the human brain. But, methods like intracranial recordings and wearable devices are becoming more important. They give new ways to look at how the brain works. These methods add to what we learn from neuroimaging.
Invasive Intracranial Recordings
Intracranial recordings let us see brain activity up close. They give us spatiotemporal resolution that’s hard to get with other methods. By putting electrodes on or in the brain, we can see how single neurons and networks work. This helps us understand how the brain does its job, especially in thinking, behavior, and diseases.
Non-Invasive Wearable Devices
Wearable devices like EEG and MEG let us study the brain in real-life settings. They’re non-invasive and can be worn by people as they go about their day. This gives us a look at how the brain works in everyday life. It’s different from lab settings but helps us understand the brain better.
Using different methods together with neuroimaging helps us learn more about the brain. Each method has its own strengths. Together, they help us understand how the brain affects our thoughts, actions, and health.
Method | Spatiotemporal Resolution | Ecological Validity | Invasiveness |
---|---|---|---|
Neuroimaging | Moderate | Low | Non-invasive |
Intracranial Recordings | High | Low | Invasive |
Wearable Devices | Moderate | High | Non-invasive |
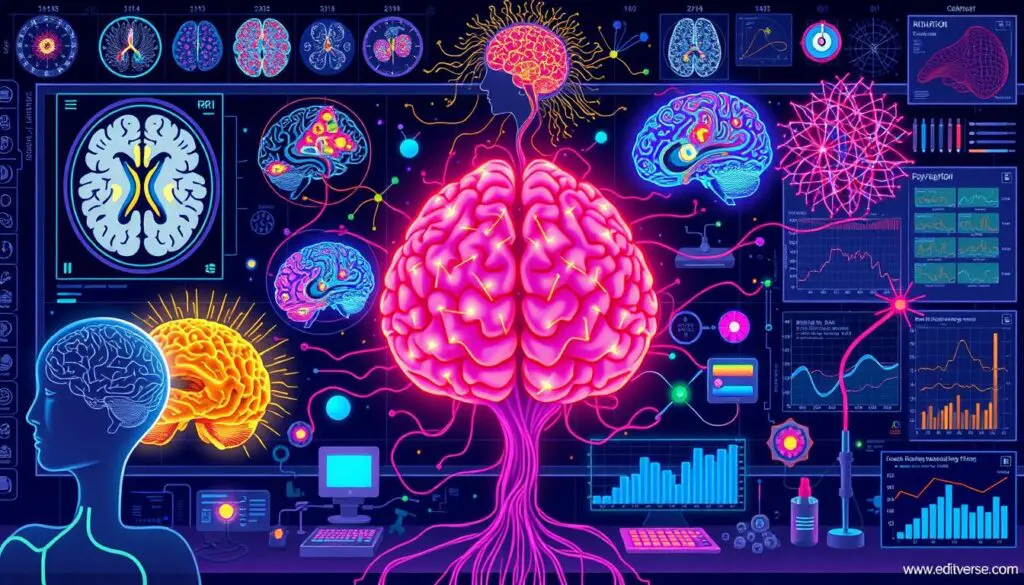
“The integration of diverse neuroscience methodologies, from neuroimaging to intracranial recordings and wearable devices, is essential for unlocking the full potential of understanding the human brain.”
Integrating Diverse Methodologies and Neurobiological Mechanisms
Today, neuroscience research needs an approach that mixes multimodal neuroimaging, computational models, and deep knowledge of neurobiological mechanisms. By using different types of data, like neuroimaging, behavior, genetics, and environmental factors, researchers can understand how the brain affects our thoughts, actions, and mental health.
Computational neuroscience and cognitive neuroscience methods give us new insights into the brain’s workings. For example, computational models can mimic brain processes and forecast how our thoughts and behaviors might change. This helps along with traditional neuroimaging techniques [1].
- New neuro-technological tools let us see brain activity closely and measure it in real life. This gives us a clearer picture of how the brain works in everyday situations.
- Using different methods, like computational models, neuroimaging, and other techniques, helps solve the “black box problem”. This is the challenge of figuring out what’s happening inside the brain, even if we know what goes in and what comes out.
By combining various neuroscience methods and sticking to strong neurobiological principles, researchers can move forward in both basic and clinical areas. This all-around approach is key to understanding the human brain better. It will help us find new ways to treat neurological and psychiatric issues.
“The integration of computational models with neuroimaging data can provide a more comprehensive understanding of the complex relationships between the brain, cognition, and behavior.”
Neuroscience, AI, and the Human Connectome Project
The Human Connectome Project is a big step forward in combining neuroscience, Artificial Intelligence (AI), and brain mapping. It uses machine learning to look into huge amounts of neuroimaging data. This helps us learn more about how the human brain works.
AI and Machine Learning in Brain Mapping
AI and machine learning are key to the Human Connectome Project. They help analyze brain activity and find new ways to understand the brain. Machine learning can even spot small differences in brain structure between men and women. This could lead to new ways to treat neurological disorders.
Neurological Insights from the Connectome Project
The Human Connectome Project is changing how we see neuroscience. It shows us how the brain works and helps us understand neurological disorders. This could lead to better treatments for these conditions.
“The Human Connectome Project represents a paradigm shift in our approach to understanding the human brain, leveraging the power of Artificial Intelligence and machine learning to uncover the complex relationships between brain structure, function, and behavior.”
Big Data Challenges in Neuroimaging and Psychiatry
Researchers in psychiatry are using big data to understand the human mind better. But, this task is tough. They face issues like the heterogeneity of mental health, many comorbidities, and complex neural connections. Big data, with its vast and complex datasets, is helping them out.
Defining Big Data in Psychiatry
In neuroimaging and psychiatry, big data offers many benefits. It boosts statistical power, makes research more generalizable, and combines different types of data. But, it also brings challenges like sharing data, standardizing it, and merging findings from various studies.
Benefits of Big Data for Psychiatric Research
- It helps find subtle effects and patterns in complex mental health data.
- It makes research findings more applicable to different people and situations.
- It combines data from neuroimaging, genetics, and clinical tests for a deeper understanding of mental health.
- It looks at common mechanisms behind different mental health issues, going beyond just the diagnosis.
As psychiatry advances, big data is changing how we see the human mind. It’s opening doors to more tailored mental health treatments. By tackling the challenges and using big data wisely, researchers can make big strides in psychiatric care.
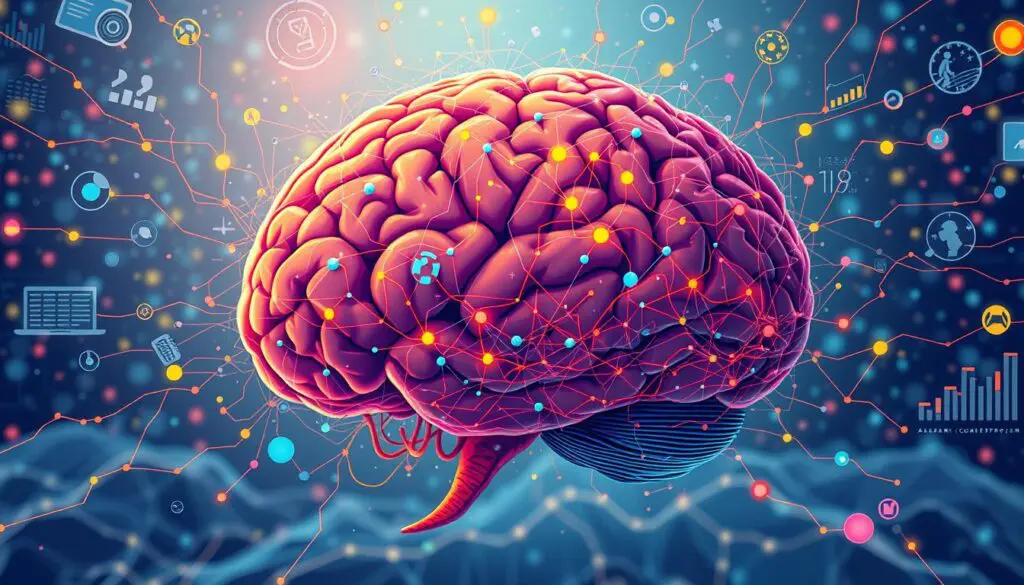
Using big data in neuroimaging and psychiatric research brings both chances and hurdles. Researchers aim to simplify the complexities of the human mind with big data. This approach could lead to more targeted and successful mental health treatments.
Best Practices for Using and Acquiring Big Data
Using big data in neuroimaging and psychiatric research is becoming more common. It’s important to know how to use and get big data right. This means understanding how to use data, managing it well, and working together to make the most of it. These steps help researchers use big data to learn more about the brain and mental health.
Working with big data means sharing and getting data right. Researchers need to know how to use big data sets like the Human Connectome Project. This lets them use the data while following the rules.
It’s also key to manage and analyze big data well. This means keeping data safe, making sure processes are the same, and using advanced tools like machine learning. Doing this makes research with big data more efficient and reliable.
Working together is another important way to use big data well. By joining forces with others, researchers can share knowledge and data. This helps solve big problems and moves the field forward.
By following these best practices, researchers can make the most of big data. They can find new insights, help patients, and learn more about the brain and mental health.
“The power of big data lies in its ability to reveal patterns, trends, and insights that were previously hidden or inaccessible. By embracing best practices in data acquisition, management, and collaboration, we can unlock the full potential of these vast resources to transform our understanding of the brain and mental health.”
Predicting Clinical Outcomes in Acute Stroke
Predicting how a stroke patient will do is a big challenge. Traditional methods don’t always work well. New studies look at using brain networks from scans to help predict outcomes. But, getting this data quickly in the early stages of stroke is hard.
Integrating Connectome Information and MRI Data
Using the Human Connectome Project and MRI can help predict stroke severity. This method could make predicting outcomes more accurate. It could lead to better treatments tailored for each patient.
Limitations of Traditional Prediction Models
Old methods for predicting stroke outcomes are not very good. Adding brain network info from scans might help more. But, getting this info fast in the early stages of stroke is tough.
Metric | Traditional Prediction Models | Integrating Connectome and MRI |
---|---|---|
Accuracy | Moderate | Improved |
Feasibility in Hyperacute Phase | High | Improved |
Personalization of Treatment | Limited | Enhanced |
“The integration of network connectivity information, derived from techniques like resting-state fMRI and diffusion tensor imaging, has shown promise in enhancing outcome prediction in acute stroke.”
Materials and Methods
We looked into how Acute Stroke, MRI, and Clinical Assessment data can predict outcomes for patients with acute or subacute single subcortical ischemic stroke. We examined the patient group and the MRI and clinical assessment methods used.
Patient Cohort and Inclusion Criteria
The study had 51 stroke patients, aged 47 to 88, with an average age of 69.0 ± 10.5. There were 24 females. They were checked at the stroke unit and intensive care unit at the University Hospital Jena, Germany. To be included, patients had to have an acute or subacute single subcortical ischemic stroke, clear neurological symptoms, an NIHSS score under 15 points at admission, and be over 18 years old. Those with other brain disorders, acute vision problems, or MRI contraindications were not included.
Clinical Assessment and MRI Protocols
Every patient got a detailed clinical check-up. This included looking at the NIHSS and mRS scores. Also, all patients had MRI scans with high-resolution T1 and FLAIR sequences within 2-5 days of being in the hospital. We used the MRI and clinical data to make predictive models for stroke patient outcomes.
“The integration of Acute Stroke, MRI, and Clinical Assessment data holds the potential to enhance our understanding of neurological recovery and inform personalized treatment strategies for stroke patients.”
Conclusion
The Human Connectome Project is a major leap forward. It combines Neuroscience, AI, and advanced brain mapping. This effort aims to understand the human brain better.
Researchers use computational models and high-resolution neuroimaging data. They’re learning about the brain’s structure, function, and connections. This could lead to new treatments for brain and mental health issues.
This project shows how Neuroscience, AI, and Brain Mapping can work together. It’s a big step for research in this area. The findings could change how we see the human mind and lead to new treatments.
The Connectome Project is a key moment in Neuroscience and AI. It shows what we can achieve when different fields work together. It opens up new ways to improve human health and well-being.
FAQ
What is the Human Connectome Project?
The Human Connectome Project is a big project to map the brain’s neural connections. It uses advanced imaging and computer models. The goal is to understand how the brain works, improve cognition, and help with neurological disorders. AI and machine learning play a big part in this.
What is computational neuroimaging?
Computational neuroimaging is a new field that mixes advanced imaging like fMRI with computer models. It studies how the brain’s structure and function relate to behavior.
What are the strengths and limitations of computational neuroimaging?
This field is great for linking brain activity to behavior and can help predict clinical outcomes. But, it’s not perfect. Some worry about how relevant lab studies are to real life. Also, some imaging methods don’t show brain activity clearly.
How does computational neuroimaging compare to other neuroscience methods?
Neuroimaging is a key tool in brain research. But, new methods like invasive recordings and wearable devices are coming up. These offer new ways to study the brain. Invasive recordings give detailed brain activity but are invasive. Wearable devices capture brain activity in real life, making research more realistic.
How can diverse neuroscience methodologies and neurobiological mechanisms be integrated?
To make the most of different research methods, we need to combine them. This means using various data types and understanding brain biology. This approach will help advance brain research and improve treatments.
How does the Human Connectome Project leverage AI and machine learning?
The Human Connectome Project uses AI and machine learning to analyze lots of brain data. This helps researchers find new insights into how the brain connects and works.
What are the challenges and benefits of using big data in neuroimaging and psychiatric research?
Studying mental health is tough because of the complexity of mental disorders. Big data can help by giving more power to research, making it more general, and looking at brain connections in new ways. But, big data also brings challenges like sharing data and combining findings.
How can big data be effectively utilized in neuroscience research?
Using big data in brain research is getting more common. It’s important to know how to use and get new data. This means following data rules, managing data well, and working together to use big datasets fully.
How can computational neuroimaging approaches help predict clinical outcomes in acute stroke?
Predicting how someone will do after a stroke is hard. Recent studies use brain network info to help predict outcomes. Another way is using data from the Human Connectome Project to predict stroke severity.
Source Links
- https://www.psypost.org/brain-connectivity-patterns-linked-to-borderline-personality-traits/
- https://brainspaceinitiative.github.io/talk-series/
- https://www.mdpi.com/2504-4990/6/3/99
- https://www.nature.com/articles/s41386-024-01946-8
- https://www.mdpi.com/2813-2475/3/3/25
- https://www.nature.com/articles/s41386-024-01973-5
- https://www.mdpi.com/1420-3049/29/17/4248
- https://www.mdpi.com/2075-5309/14/9/2816
- https://www.mdpi.com/2073-4409/13/17/1498
- https://scholaridea.com/2024/09/04/102-postdoctoral-scholarships-at-harvard-university-united-states/
- https://www.brainfacts.org/in-the-lab/tools-and-techniques/2024/ai-targets-location-where-female-and-male-brains-differ-081324
- https://www.nature.com/articles/s41586-024-07805-2
- https://dornsife.usc.edu/news/stories/presidential-debate-questions-for-donald-trump-and-kamala-harris/
- https://www.mdpi.com/2072-6643/16/17/3026
- https://www.mdpi.com/2304-8158/13/17/2841
- https://www.mdpi.com/2072-6643/16/17/3018
- https://www.mdpi.com/1099-4300/26/9/767
- https://www.mdpi.com/1422-0067/25/17/9698
- https://www.mdpi.com/2223-7747/13/17/2516
- https://www.mdpi.com/2076-3417/14/17/8011
- https://www.mdpi.com/2079-7737/13/9/703