Did you know over 70 percent of major epidemiology research uses advanced biostatistics? As health data grows fast, we need better analytical tools. This need for precise data analysis is key to today’s health efforts.
Epidemiology now leans on advanced biostatistics to handle complex data. These methods are key for predicting disease outbreaks and understanding health genetics. Today’s top studies use genetics, genomic analysis, and predictive models for deep insights.
The QuantGen group, led by Drs. Rohan de los Campos and Paulino Vazquez, shows how these methods work together. They’ve created software like BGLR and BGData for big genomic data. Their work is crucial in the Enigma consortium, focusing on genetics and mental health risks.
Key Takeaways
- The demand for advanced biostatistical methods in public health research is skyrocketing.
- Quantitative genetics, genomic analysis, and predictive model development are pivotal in contemporary epidemiology.
- QuantGen group’s contributions are vital to modern genomic data handling techniques.
- Software such as BGLR and BGData exemplify the forefront of biostatistical tools.
- The Enigma consortium’s ORIGINs group leverages these methods to study early brain development and psychiatric diseases.
Introduction to Advanced Biostatistical Methods in Epidemiology
Advanced biostatistical methods are key in epidemiology. They provide complex analytical tools and models for health data. Courses like FMPH 102 – Biostatistics in Public Health teach students about biostatistical analysis. Topics include probability, statistical distributions, hypothesis testing, and linear regression.
Students also learn about specialized areas. For example, EPID 5260 Biostatistics for Epidemiologic Methods I and EPID 6250 Advanced Biostatistical Methods for Multivariable Prediction Models focus on predictive modeling in *epidemiological research methods*. EPID 6300 Clinical Trials highlights the role of experimental research in epidemiology.
Other courses, like EPID 5370 Data Management and Visualization II, teach students how to handle and interpret data. This is crucial for public health data interpretation. EPID 6750 Advanced Methods for Analysis of Complex Genetic Traits combines genetic epidemiology with biostatistics. This helps epidemiologists analyze complex traits better.
Course Code | Title | Units | Key Areas |
---|---|---|---|
FMPH 40 | Introduction to Public Health | 4 | Infrastructure, Analytical Tools, Health Promotion |
FMPH 101 | Epidemiology | 4 | Basic Principles, Disease Investigation |
FMPH 102 | Biostatistics in Public Health | 4 | Probability, Hypothesis Testing, Linear Regression |
EPID 5260 | Biostatistics for Epidemiologic Methods | 4 | Predictive Modeling, Multivariable Analysis |
EPID 6750 | Advanced Methods for Analysis of Complex Genetic Traits | 4 | Genetic Epidemiology, Data Interpretation |
EPID 6300 | Clinical Trials | 4 | Experimental Research Methods |
By taking these challenging courses and doing practical exercises, students get ready to improve public health interventions and policy. They learn to use advanced biostatistical methods for public health data interpretation. This thorough education prepares the next generation of epidemiologists to face complex health issues.
Epidemiological Data Analysis Techniques
In epidemiology, data analysis is key to understanding health patterns. Regression methods, especially for case-control studies, are crucial. They help find links between risk factors and health outcomes.
Regression Methods for Case-Control Studies
For case-control studies, logistic regression and biostatistical modeling are vital. They help researchers understand how exposures affect health outcomes.
- Unconditional Logistic Regression: This method helps control for many confounding variables. For example, in Palermo, it was used to study lifestyle and mental health in 5444 undergraduates, adjusting for demographics.
- Conditional Logistic Regression: This is great for matched case-control studies. A study of 10,530 Belgian workers looked at how job changes affected health, controlling for age and gender.
Using biostatistical modeling in these studies ensures the data is analyzed carefully. A study in Italy on TB infection in healthcare students used this method to adjust for health factors.
As research advances, combining logistic regression with spatial-temporal modeling is key. This was seen in Pavia, where PM2.5 exposure risks were assessed. This approach looks at both time and place in disease risk factors.
“The combination of case-control study analysis, logistic regression, and biostatistical modeling in epidemiology helps us understand disease associations and risk factors. This mix of techniques brings precision and detail to interpreting data, helping in disease prevention and control.”
Study Type | Location | Participants | Focus |
---|---|---|---|
Cross-sectional | Palermo, Italy | 5444 undergraduates | Lifestyle and psychological well-being |
Retrospective longitudinal | Belgium | 10,530 employees | Employment change and work-related diseases |
Cohort | Italy | Healthcare students | Latent tuberculosis infection |
Statistical Modeling for Public Health
Statistical modeling is key in public health. It helps experts predict health outcomes from different data sets. By using past and current data, these models spot risk factors. This helps in making better health interventions.
Courses and tools in this area teach professionals to analyze and understand data well.
Development of Predictive Models
Creating predictive models in public health starts with courses like PUBHLTH 223. This course teaches basic skills in organizing and summarizing data. Then, PUBHLTH 460 goes deeper, working with complex data and programming.
These courses are the base for learning the stats needed for forecasting health outcomes and public health stats. PUBHLTH 490Z and PUBHLTH 690Z take it further, offering hands-on experience with advanced methods like linear and logistic regression, and machine learning. This training helps professionals build strong predictive models to spot and prevent health risks.
Practical Applications
Predictive analytics in public health has many uses. For example, PUBHLTH 690Z teaches how to turn scientific questions into statistical frameworks using R programming. PUBHLTH 730 focuses on Bayesian statistical modeling for complex health data.
These advanced courses help public health experts design and analyze cluster-randomized trials. This leads to health strategies based on solid evidence.
Look at the practicum topics in the Epidemiology and Biostatistics Functional Certificate. They include risk prediction models for carotid endarterectomy patients and studies on chronic kidney disease epidemics. These projects show how statistical modeling tackles real-world health issues.
Course | Focus Area | Key Skills |
---|---|---|
PUBHLTH 223 | Basic Data Analysis | Organizing and Summarizing Data |
PUBHLTH 460 | Complex Data Relationships | Linear and Logistic Regression, R Programming |
PUBHLTH 490Z | Statistical Modeling | Multiple Linear Regression, Machine Learning |
PBHL –B 572 | Categorical Data Analysis | Binary, Multilevel, and Count Data Analysis |
PUBHLTH 690Z | Health Data Science | Analytics using Real Health Data |
EP850 | Intermediate Epidemiology | Epidemiological Data Analysis using SAS |
To lead in public health, it’s important to blend knowledge from these courses with advanced methods like spatial modeling and GIS. This helps spot spatial patterns and understand environmental risks. Making informed decisions is key to better health outcomes for all.
Survival Analysis Techniques in Epidemiology
Survival analysis is key in epidemiology. It helps researchers study time-to-event data. This is important for understanding health outcomes.
Introduction to Cox Proportional Hazards Model
The Cox Proportional Hazards Model is a vital tool in survival analysis. It lets researchers see how risk factors affect the time to an event, like getting a disease or dying. The model can look at many variables at once, giving a detailed view of health outcomes.
The Cox model relies on the assumption of proportional hazards. This means the risk stays the same over time. This is crucial in understanding risk factors. The model is a key tool in survival analysis, helping us see what affects health trends.
Advanced Methods
Advanced survival analysis builds on the Cox model. It helps find complex relationships in data. Techniques like time-varying covariates and frailty models make risk analysis more detailed.
The University of Washington’s Survival Analysis Techniques course shows this advanced approach. It covers everything from basic to complex biostatistics. Students learn a lot, from descriptive stats to logistic regressions.
The course is structured as follows:
Course Component | Weight |
---|---|
Examinations | 50% |
Homework | 25% |
Data Analysis Project | 25% |
Late assignments lose 20 percentage points each day. The course values regular attendance and helps students with disabilities. Led by experts like Bertram K. C. (Bert) Chan, PhD, it uses R to teach biostatistics. This makes students more analytical.
Longitudinal Data Analysis
Longitudinal data analysis helps us see how diseases change over time. It involves collecting data from the same people over several periods. This method, known as a longitudinal study, shows us changes, growth, and patterns. It helps us understand public health issues better, leading to better strategies to fight them.
Biostatistical computations are key to doing longitudinal studies well. Modern stats like the mixed effect regression model help manage complex data and missing values. These models are great for handling tough data challenges and are used in studies and trials.
There are various ways to analyze longitudinal data. Techniques like Change Score Analysis and Repeated Measures ANOVA are used. Others include Generalized Estimating Equations and Mixed Effect Regression models. These methods help with tricky data like uneven timing and changing predictors.
Here’s a quick look at how cross-sectional and longitudinal studies differ:
Study Type | Variability | Statistical Power |
---|---|---|
Cross-Sectional Study | Higher | Lower |
Longitudinal Study | Lower | Higher |
For example, studies on Huntington’s disease use longitudinal methods. They look at how treatments affect motor, cognitive, and mental health over time. This deep look helps spot patterns for better treatments.
In summary, strong biostatistical methods and thorough data tracking are key in longitudinal studies. These studies are vital in epidemiology. They help us understand how diseases progress and how well health measures work.
Bayesian Methods in Epidemiology
Bayesian methods are changing epidemiology with a new way of looking at data. They mix what we already know with the unknown to make predictions more accurate. This helps in understanding risks better and making predictions.
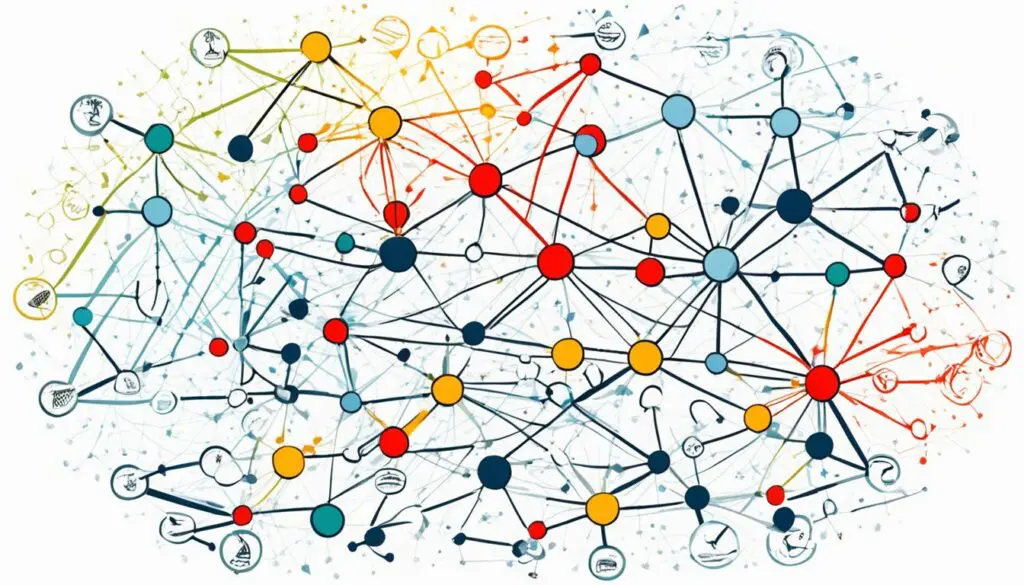
Bayesian Inference and Its Applications
Bayesian inference is key in many areas of epidemiology. It helps make models and tools for predicting risks. For instance, the BGLR package is a big help in complex data analysis, thanks to NIH funding.
Tools like Pedigreemm and pedigreeTools make genetic studies more precise. They work with DNA data to predict traits and understand families better.
Software for Bayesian Analysis
There are many tools for Bayesian methods in epidemiology. The BGLR package and MTM functionality are great for complex genetic studies. Pleiotest is another tool for big data sets.
Pedigreemm and MOSS help with mixed models and combining different types of data. These tools are vital for projects like ORIGINS, which aims to predict cognitive abilities.
Together, these tools make Bayesian methods easier to use in epidemiology. They help make decisions in public health based on solid evidence.
Causal Inference in Observational Studies
Observational studies are key for understanding causal relationships in epidemiology. They help researchers tell apart mere links and true causes. This is vital for making solid conclusions and shaping health policy.
“Counterfactualism has become the de facto school of thought for epidemiology, attempting to replicate the counterfactual condition between exposure groups.”
To deeply explore these relationships, many advanced methods are used. These include traditional regression, G-computation, propensity scoring, and structural equation modeling. These methods tackle confounding factors and more.
It’s important to consider bias, errors, and missing data for better data interpretation. Some methods aim to mimic randomized controlled trials. These trials are known for their strong evidence on causality.
Method | Focus |
---|---|
Traditional Regression | Controlling confounding factors |
G-Computation | Estimating causal effects |
Propensity Scoring | Balancing exposure groups |
Structural Equation Modeling | Modeling complex relationships |
Practitioners play a big role in making sure solutions are practical. As these methods get better, they help us understand causes better. This also helps in making better health policies.
Courses and workshops, like those from CAUSALab, are great for learning these methods. The next one in Boston, Massachusetts, from June 3 to June 14, 2024, will focus on important topics. Remember, registration started on February 7, 2024, and ends on May 27, 2024.
Multilevel and Hierarchical Models
Multilevel and hierarchical models are key in handling complex data in epidemiology. They recognize the layered nature of data, like patients in hospitals or kids in schools. This method is vital for precise analysis in health research.
Nested Data Structures
Looking into nested data helps us deal with group correlations, a big challenge in health studies. This method tackles the clustering effect, which is often seen in data. For example, a 2004 study by Raudenbush and Bryk showed how analyzing hierarchical data can reveal group-level effects.
Applications in Public Health
Multilevel modeling is very useful in public health. It helps us understand how health interventions work across different levels. A 2007 study by Wright and Subramanian used multilevel analysis to study asthma disparities, showing the power of these methods.
Also, spatial analysis is a big part of multilevel models. It’s key for seeing health patterns and targeting interventions. For more on this, check out spatial analysis in public health.
When setting up multilevel models, think about things like sample size and how groups relate to each other. Useful guides like the multilevel modeling guidelines can help. They give tips for better analysis, including how to handle outliers, as explained by Finch in 2017.
Spatial Epidemiology and Disease Mapping
Spatial epidemiology looks at how places affect health and disease spread. It uses disease mapping and spatial data analysis to see where health issues happen. Health geography is key in spotting these patterns. This helps plan health actions and strategies.
Knowing where diseases spread helps us understand what causes them. This lets us guess where diseases might strike next. With this info, we can focus health efforts where they’re most needed.
Spatial data analysis links health to geographic factors. It’s vital for finding disease clusters, checking out the environment, and deciding where to use resources. It also helps make predictions to improve disease tracking and control.
Learning about health geography helps spot disease hotspots. It lets health experts make interventions that fit the area’s needs. With tools like GIS, they can make maps that show health trends clearly.
- Visualizing disease distribution through mapping techniques.
- Analyzing spatial variations to detect clusters and trends.
- Implementing targeted public health interventions.
Course | Focus Area |
---|---|
BIOS 700 | Descriptive statistics, probability, and hypothesis testing specific to health data. |
BIOS 701 | Advanced statistical applications to public health, including measures of association. |
BIOS 709 | Effective data storage, cleaning, merging, and formatting using statistical software. |
BIOS 710 | Advanced techniques in statistical data management. |
BIOS 746 | Linear, logistic, and survival analysis models for complex survey data. |
Advanced Biostatistical Methods in Epidemiology
Epidemiology has changed a lot with new methods. Now, we use advanced techniques to analyze complex data. This includes looking at many variables at once and combining different types of data. Thanks to this, we can better understand health trends.
Ph.D. students can bring up to eight courses for credit. If you want to join the Biostatistics program, apply through SOPHAS. On average, about four students join each class.
The Brown University Department of Biostatistics has a team of experts. They work on topics like spatial data, learning from data, bioinformatics, and models for different levels. This work helps us understand health better.
To get into a Ph.D. program, you need to show you’re good in a specific area like genetics or epidemiology. Students must take at least five courses, including two in biostatistics and one in another field. Luckily, all Ph.D. students get full aid, covering tuition, stipend, and health insurance.
Advanced courses are key for learning about statistics in public health. They teach us about complex statistical methods. Students learn about regression and deep epidemiological studies. This focus on practical skills and electives prepares graduates well.
Conclusion
The blend of epidemiology, biostatistics, and data science has changed how we analyze health data, especially with electronic medical records (EMRs). This new era uses advanced biostatistical methods that have been improved over the last 20 years. These methods are now a key part of what medical students learn.
Medical students get about 50 hours of training in this field. This matches the standards set by the National Board of Medical Examiners. The course covers important topics that are tested in the USMLE Step 1.
The future of epidemiological studies is closely linked to ongoing biostatistical advancements. New methods like Targeted Maximum Likelihood Estimation (TMLE) and better ways to design studies make research more efficient and thorough. These advances are key for making policies based on solid evidence, helping with emergency planning, disease control, and health policy.
As we dive deeper into the age of big data, using FAIR (Findable, Accessible, Interoperable, Reusable) practices helps researchers work together better. This leads to more impactful epidemiological research. By using advanced analytical tools and following the latest best practices, we can keep improving public health efforts.
This approach combines epidemiology, biostatistics, and data science to tackle complex health issues. It leads to big improvements in health outcomes worldwide. This shows how important advanced biostatistical methods are.
FAQ
What are Advanced Biostatistical Methods in Epidemiology?
How can biostatistical methods help in case-control studies?
What is the significance of predictive models in public health statistics?
How does the Cox Proportional Hazards Model aid in survival analysis?
What is the importance of longitudinal data analysis in epidemiology?
How are Bayesian methods applied in epidemiology?
What role do observational studies play in understanding causal relationships in epidemiology?
How do multilevel and hierarchical models contribute to public health analysis?
What is spatial epidemiology and how does it use disease mapping?
Why are advanced biostatistical methods crucial in epidemiology?
Source Links
- https://www.umu.se/en/education/courses/advanced-biostatistics-and-epidemiology2/ – Advanced biostatistics and epidemiology
- https://ysph.yale.edu/school-of-public-health/graduate-programs/accelerated-mph-program/applied-biostatistics-and-epidemiology/ – Advanced MPH Applied Biostatistics & Epidemiology | Yale School of Public Health
- https://catalog.ucsd.edu/courses/FMPH.html – Public Health
- https://epibiostat.ucsf.edu/individual-courses – Individual Courses
- https://catalog.upenn.edu/courses/epid/ – Epidemiology (EPID) < University of Pennsylvania
- https://www.ncbi.nlm.nih.gov/pmc/articles/PMC8036932/ – Statistical Advances in Epidemiology and Public Health
- https://ebooks.inflibnet.ac.in/antp13/chapter/biostatistics-epidemiologic-method/ – 14 Biostatistics & Epidemiologic Method
- https://www.ncbi.nlm.nih.gov/pmc/articles/PMC2853157/ – Data Analysis of Epidemiological Studies: Part 11 of a Series on Evaluation of Scientific Publications
- https://www.umass.edu/public-health-sciences/academics/biostatistics/course-descriptions – Biostatistics Course Descriptions
- https://www.bu.edu/sph/education/degrees-and-programs/master-of-public-health-programs/certificates/mph-functional-certificates/epidemiology-and-biostatistics/ – Epidemiology and Biostatistics | SPH
- https://bulletins.iu.edu/iu/gradschool/2021-2022/programs/indianapolis/biostatistics/courses.shtml – Courses : Academic Bulletin
- https://www.biostat.washington.edu/sites/default/files/2020-01/2019_WIN_BIOST_537_CaroneM.pdf – Microsoft Word – syllabus_537_2019.docx
- https://www.springerpub.com/biostatistics-for-epidemiology-and-public-health-using-r-9780826110251.html – Biostatistics for Epidemiology and Public Health Using R
- https://www.ncbi.nlm.nih.gov/pmc/articles/PMC5633048/ – Statistical Approaches to Longitudinal Data Analysis in Neurodegenerative Diseases: Huntington’s Disease as a Model
- https://publichealth.jhu.edu/academics/graduate-summer-institute-of-epidemiology-and-biostatistics/courses – Courses | Johns Hopkins Bloomberg School of Public Health
- https://www.cceb.med.upenn.edu/course-descriptions – Course Descriptions | CCEB
- https://epibio.msu.edu/research/abm – Advanced Biostatistical Methods | Department of EPIDEMIOLOGY AND BIOSTATISTICS
- https://link.springer.com/10.1007/978-0-387-09834-0_57 – Bayesian Methods in Epidemiology
- https://epibiostat.ucsf.edu/epidemiologic-methods-ii-epi-207 – Epidemiologic Methods II (EPI 207)
- https://www.goldsteinepi.com/blog/notes-on-causal-inference-in-observational-epidemiology/index.html – Neal D. Goldstein, PhD, MBI
- https://causalab.sph.harvard.edu/courses/ – 2024 Causal Courses
- https://www.ncbi.nlm.nih.gov/pmc/articles/PMC10942821/ – Application of Hierarchical/Multilevel Models and Quality of Reporting (2010–2020): A Systematic Review
- https://academic.oup.com/ije/article/29/1/158/666823 – Principles of multilevel modelling
- https://bulletin.temple.edu/graduate/courses/epbi/ – Epidemiology and Biostatistics (EPBI) < Temple University
- https://academicbulletins.sc.edu/graduate/course-descriptions/bios/ – Biostatistics (BIOS) < University of South Carolina
- https://academic.oup.com/book/8189/chapter/153708629 – Spatial epidemiology: methods and applications | Spatial Epidemiology: Methods and Applications
- https://biostatistics.sph.brown.edu/academics/doctoral-program-biostatistics – Doctoral Program in Biostatistics
- https://www.publichealth.columbia.edu/academics/degrees/master-public-health/certificates/advanced-epidemiology – Advanced Epidemiology
- https://sph.cuny.edu/academics/degrees-and-programs/masters-programs/mph-in-epidemiology-and-biostatistics/ – MPH in Epidemiology and Biostatistics – CUNY Graduate School of Public Health & Health Policy
- https://www.iamse.org/mse-article/commentary-techniques-for-effective-teaching-of-a-medical-school-course-on-biostatistics-and-epidemiology/ – COMMENTARY: Techniques for Effective Teaching of a Medical School Course on Biostatistics and Epidemiology – International Association of Medical Science Educators – IAMSE
- https://wjarr.com/sites/default/files/WJARR-2024-0178.pdf – Epidemiological statistical methods: A comparative review of their implementation in public health studies in the USA and Africa
- https://www.academia.edu/27948372/Biostatistical_Methods_in_Epidemiology – Biostatistical Methods in Epidemiology